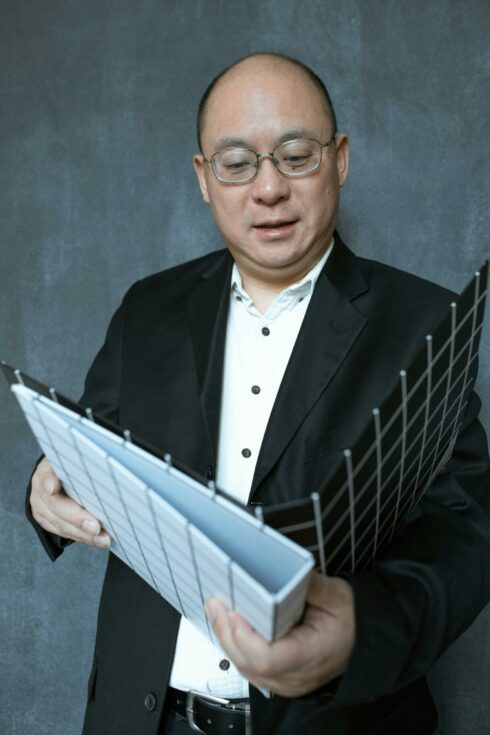
Organizations are getting caught up within the hype cycle of AI and generative AI, however in so many circumstances, they don’t have the info basis wanted to execute AI tasks. A 3rd of executives assume that lower than 50% of their group’s information is consumable, emphasizing the truth that many organizations aren’t ready for AI.
For that reason, it’s vital to put the appropriate groundwork earlier than embarking on an AI initiative. As you assess your readiness, listed below are the first concerns:
- Availability: The place is your information?
- Catalog: How will you doc and harmonize your information?
- High quality: Having good high quality information is essential to the success of your AI initiatives.
AI underscores the rubbish in, rubbish out downside: should you enter information into the AI mannequin that’s poor-quality, inaccurate or irrelevant, your output shall be, too. These tasks are far too concerned and costly, and the stakes are too excessive, to start out off on the incorrect information foot.
The significance of knowledge for AI
Knowledge is AI’s stock-in-trade; it’s skilled on information after which processes information for a designed function. Whenever you’re planning to make use of AI to assist clear up an issue – even when utilizing an current giant language mannequin, akin to a generative AI software like ChatGPT – you’ll have to feed it the appropriate context for what you are promoting (i.e. good information,) to tailor the solutions for what you are promoting context (e.g. for retrieval-augmented technology). It’s not merely a matter of dumping information right into a mannequin.
And should you’re constructing a brand new mannequin, you must know what information you’ll use to coach it and validate it. That information must be separated out so you may prepare it towards a dataset after which validate towards a special dataset and decide if it’s working.
Challenges to establishing the appropriate information basis
For a lot of corporations, understanding the place their information is and the provision of that information is the primary massive problem. If you have already got some degree of understanding of your information – what information exists, what techniques it exists in, what the foundations are for that information and so forth – that’s a superb place to begin. The actual fact is, although, that many corporations don’t have this degree of understanding.
Knowledge isn’t all the time available; it could be residing in lots of techniques and silos. Massive corporations specifically are inclined to have very difficult information landscapes. They don’t have a single, curated database the place every part that the mannequin wants is properly organized in rows and columns the place they’ll simply retrieve it and use it.
One other problem is that the info isn’t just in many alternative techniques however in many alternative codecs. There are SQL databases, NoSQL databases, graph databases, information lakes, generally information can solely be accessed by way of proprietary software APIs. There’s structured information, and there’s unstructured information. There’s some information sitting in information, and possibly some is coming out of your factories’ sensors in actual time, and so forth. Relying on what trade you’re in, your information can come from a plethora of various techniques and codecs. Harmonizing that information is troublesome; most organizations don’t have the instruments or techniques to try this.
Even when yow will discover your information and put it into one widespread format (canonical mannequin) that the enterprise understands, now you must take into consideration information high quality. Knowledge is messy; it could look nice from a distance, however whenever you take a better look, this information has errors and duplications since you’re getting it from a number of techniques and inconsistencies are inevitable. You’ll be able to’t feed the AI with coaching information that’s of low high quality and anticipate high-quality outcomes.
How one can lay the appropriate basis: Three steps to success
The primary brick of the AI mission’s basis is understanding your information. You have to have the flexibility to articulate what information what you are promoting is capturing, what techniques it’s residing in, the way it’s bodily applied versus the enterprise’s logical definition of it, what the enterprise guidelines for it are..
Subsequent, you need to have the ability to consider your information. That comes all the way down to asking, “What does good information for my enterprise imply?” You want a definition for what good high quality seems like, and also you want guidelines in place for validating and cleaning it, and a technique for sustaining the standard over its lifecycle.
In the event you’re capable of get the info in a canonical mannequin from heterogeneous techniques and also you wrangle with it to enhance the standard, you continue to have to handle scalability. That is the third foundational step. Many fashions require numerous information to coach them; you additionally want plenty of information for retrieval-augmented technology, which is a way for enhancing generative AI fashions utilizing data obtained from exterior sources that weren’t included in coaching the mannequin. And all of this information is repeatedly altering and evolving.
You want a technique for learn how to create the appropriate information pipeline that scales to deal with the load and quantity of the info you would possibly feed into it. Initially, you’re so slowed down by determining the place to get the info from, learn how to clear it and so forth that you just may not have absolutely thought by means of how difficult it will likely be whenever you attempt to scale it with repeatedly evolving information. So, you must contemplate what platform you’re utilizing to construct this mission in order that that platform is ready to then scale as much as the amount of knowledge that you just’ll carry into it.
Creating the surroundings for reliable information
When engaged on an AI mission, treating information as an afterthought is a positive recipe for poor enterprise outcomes. Anybody who’s critical about constructing and sustaining a enterprise edge by creating and utilizing AI should begin with the info first. The complexity and the problem of cataloging and readying the info for use for enterprise functions is a big concern, particularly as a result of time is of the essence. That’s why you don’t have time to do it incorrect; a platform and methodology that allow you to keep high-quality information is foundational. Perceive and consider your information, then plan for scalability, and you may be in your method to higher enterprise outcomes.