Introduction
In as we speak’s digital period, the facility of information is plain, and those that possess the talents to harness its potential are main the cost in shaping the way forward for know-how. Amongst these trailblazers stands an distinctive particular person, Mr. Nirmal, a visionary within the realm of information science, who has risen to grow to be a driving drive at one of many world’s foremost know-how giants, working as Microsoft’s Senior Knowledge Scientist.
Meet Mr. Nirmal, the embodiment of perseverance, brilliance, and unwavering dedication. From humble beginnings, Mr. Nirmal launched into a transformative journey that led them to the head of their profession as a Senior Knowledge Scientist at Microsoft. His meteoric rise serves as an inspiring success story, not just for aspiring information scientists however for anybody with a dream and the dedication to realize greatness.
On this success story article, we delve deep into Mr. Nirmal’s journey, tracing the important thing milestones, challenges, and triumphs which have formed their extraordinary profession. We discover the groundbreaking initiatives he has led, the transformative influence he made, and the invaluable classes he discovered alongside the way in which. Via Mr. Nirmal’s story, we uncover the traits and mindset essential to thrive within the ever-evolving world of information science.
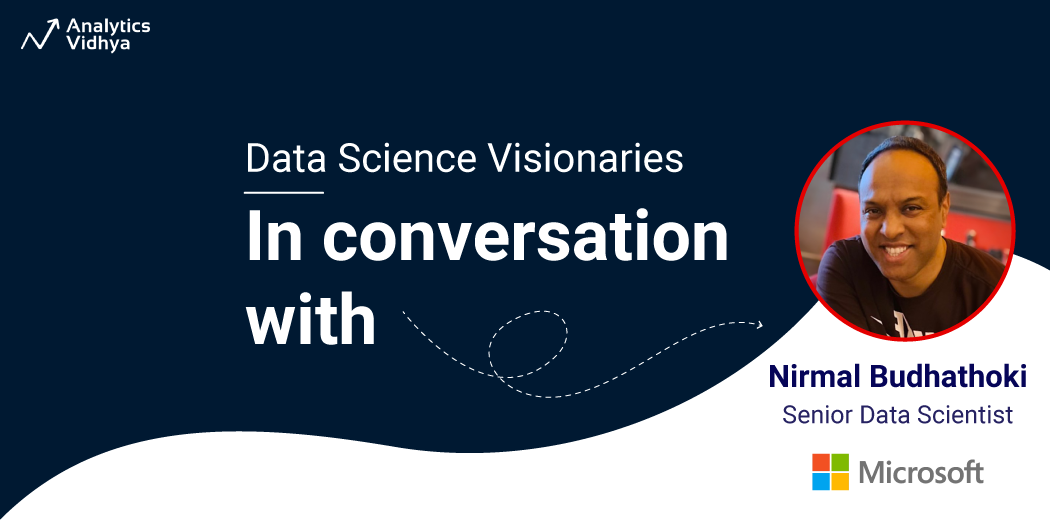
Let’s Start with the Dialog!
AV: Please spotlight your profession trajectory, academic background, and the way did it allow you to get your first information scientist job?
Mr. Nirmal: My profession trajectory has by no means been a linear path. All of us have our personal tales, and I’m positive all of them are fascinating. Right here is mine: I accomplished my Undergrad in IT Engineering from Nepal. I moved to the USA in 2007 for my Masters Diploma. After finishing my Grasp’s, I joined the US Military. Sure, it sounds very unusual. Due to the nice recession within the US round 2009 (which additionally occurred to be my commencement 12 months), the job market was very unhealthy, particularly for worldwide college students. There was a particular pilot program run by the US Military, and I went by means of all of the required processes to grow to be a service member. Rising up, I had some ardour to affix the army. What a technique to fulfill that.
Whereas I used to be within the army, I accomplished my MBA. In 2014, after my first enlistment contract was accomplished, I left the US Military. In the identical 12 months, I obtained my first information position as a Cyber Safety Analyst, working as a US federal authorities worker for the Division of Navy. I accomplished my third Masters in Knowledge Science whereas I used to be engaged on this job. After gaining some expertise working as a Knowledge Analyst, and constructing the educational credentials plus expertise on Knowledge Science, I transitioned to the personal trade taking my first position as a Knowledge Scientist title for Wells Fargo Financial institution in 2018. Since then I’ve been in information science, and presently working as Senior Knowledge Scientist for Microsoft.
AV: Are you able to inform us a few undertaking you labored on the place you had to make use of information to resolve a real-world downside and the influence it had on the enterprise or product technique?
Mr. Nirmal: There are various examples. Initially, we would not have to carry a ‘Knowledge Scientist’ title to work and remedy any information issues. There are some misconceptions like that. We may be working as Knowledge Analysts, Knowledge Engineers, Enterprise Analysts or any titles working with information.
I largely work within the cyber safety area. Two of the foremost focus areas for us are: investigation and detection. When coping with cyber safety issues, one of many very talked-about downside areas is anomaly detection. I’ve labored in a knowledge science staff to construct anomaly detention techniques, serving to the safety analysts save time on what occasions/alerts to concentrate on. The influence is on saving their time and sources.
AV: What was essentially the most difficult downside you may have solved utilizing information science? How did you strategy the issue? What was the end result?
Mr. Nirmal: I might say – essentially the most difficult downside for me is but to be solved. As we reside on the planet of extremely progressive AI, we must always all the time bear in mind that adversaries now have essentially the most superior instruments than ever. Nevertheless if I’ve to say one fascinating downside then I might decide the person conduct evaluation or additionally known as person entity conduct evaluation , broadly referred to as UEBA within the trade. UEBA is a sort of cybersecurity function that discovers the threats by figuring out person exercise that deviates from a traditional baseline.
One easy instance: We now have a person who typically logins from location A, and unexpectedly we see login exercise from location B. This may very well be regular associated to journey, however it’s nonetheless deviation from the conventional conduct so should be checked out to verify normality vs. maliciousness. Probably the most difficult a part of UEBA is to grasp and create the baseline.
Knowledge-driven Insights
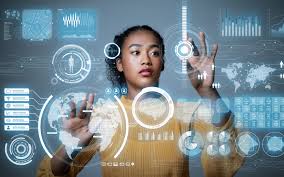
AV: May you share a narrative a few time if you needed to talk complicated data-driven insights to non-technical stakeholders? How did you be certain that they understood the insights and the influence that they had on the enterprise?
Mr. Nirmal: As a knowledge scientist, we are going to come throughout a number of situations like these. A lot of the enterprise stakeholders are effectively versed with their downside and meant options. Nevertheless typically it’s exhausting to elucidate to them why some options make sense and why some don’t. I can share one instance. We constructed a fraud detection mannequin, it was a binary classifier with fraud vs. non fraud transactions. The fraud analysts know their area effectively. However for us to elucidate the mannequin outcomes again to them was difficult to interrupt it down into their language.
If we share particulars like – mannequin tuning and hyper parameters or cross validation or sampling strategies, these items will make much less sense to them. Nevertheless if we interpret into increased ranges like what attributes we discovered helpful primarily based on the function rating, what are some challenges with lessons being imbalanced, these issues will make sense to them. Subsequently it’s all the time essential for a knowledge scientist to speak in enterprise language as effectively.
AV: How do you make sure that the machine studying fashions your staff builds are explainable and clear to the end-users, significantly within the context of safety and menace detection?
Mr. Nirmal: Like I discussed in a earlier instance, mannequin interoperability is essential with regards to explaining it again to the enterprise companions. That is essential no matter which area you’re working. In safety and menace detection, it turns into extra essential as a result of something we construct as a mannequin, shall be explainable to the menace analysts to allow them to take acceptable actions. One good instance that I can share right here is the idea of Benign Constructive. Once I first heard about this time period, I used to be a bit of confused, as I used to be solely conscious of true positives, and false positives. However within the safety area, benign positives are essential. Right here is the breakdown of these classes:
- True constructive (TP): A malicious motion detected by a safety device.
- Benign true constructive (B-TP): An motion detected by a safety device that’s actual, however not malicious, equivalent to a penetration take a look at or identified exercise generated by an permitted software.
- False constructive (FP): A false alarm, which means the exercise didn’t occur.
AV: Have you ever ever encountered a state of affairs the place the information you have been working with was messy or incomplete? How did you deal with it, and what was the end result?
Mr. Nirmal: This occurs on a regular basis. If a knowledge scientist says he/ she obtained clear information to work with, then that will probably be like a lottery ticket successful for him/her. Actual world initiatives usually are not just like the Kaggle competitors the place information comes largely clear as csv recordsdata. We spend extra time on information wants, working with information homeowners for information contract, information assortment. These are the issues that come even earlier than the exploratory information evaluation (EDA) occurs.
More often than not, we encounter messy information with some discrepancies with schema. Knowledge versioning is essential, the place we hold observe of every model of information after we iterate a number of instances to orchestrate the ETL pipeline till we get the proper information. There’s a idea of information observability which suggests precisely the identical as I discussed right here. It offers with getting the proper information to the proper locations, in the proper codecs, on the proper time.
AV: Are you able to inform us a few undertaking the place you collaborated with a staff to realize a standard purpose? How did you contribute to the staff’s success? What did you study from the expertise?
Mr. Nirmal: In Microsoft, we comply with one thing known as ‘One Microsoft’, which focuses on growing providers and merchandise that can embrace the tradition of collaboration throughout the groups to innovate novel ideas and work on it collectively , relatively than working in siloed methods. Virtually all of the initiatives that I’ve labored on are in collaboration with different teams- which may very well be engineering counterparts, or exterior groups. One benefit of Microsoft’s tradition is- they make us concentrate on constructing techniques on prime of current providers, relatively than re-inventing the wheels. This not solely promotes constructing relationships with different groups, but additionally saves time and sources for the corporate. Personally I’ve discovered many issues working with totally different groups.
Knowledge Safety Initiatives
AV: You talked about that you just love working on the intersection of safety and information science. May you share successful story a few undertaking the place you used information to enhance safety measures or forestall safety breaches? What was the influence of the undertaking?
Mr. Nirmal: This can be a nice query. Thanks for bringing it up. Since information is all over the place, information science turns into relevant for all domains. I often counsel the early profession information scientists to strive a number of paths, atleast have three domains of curiosity so you are able to do trial and error, identical to coaching machine studying fashions, profession path choice is an iterative course of to start with of your profession. Safety and information science is likely one of the uncommon and distinctive combos. The job market is in demand, and within the harsh economic system, job safety can also be stronger on this area.
To share my story, probably the greatest issues for me being in safety is that it’s a continuously evolving subject. Hackers are arising with new methods and instruments, and we’ve to reply to that very quickly. One of many easy and but useful initiatives from a enterprise standpoint, that I used to be a part of is – Alerts Classification. Because the safety researchers discover varied assault patterns, they assist safety engineers write detection guidelines, which in flip fires alerts if there’s a match or hit with the foundations. Nevertheless the issue is that each system generates hundreds of occasions that are transformed to alerts. The false constructive charge on these alerts are excessive.
To stability safety and effectivity, we developed an ML mannequin to categorize alerts into true positives, benign positives, and false positives, ranked by threat scores. This permits analysts to prioritize their queues and keep away from overwhelming volumes of alerts whereas minimizing the chance of adversaries slipping by means of undetected.
Recommendation on Dealing with Sudden Insights
AV: Have you ever ever encountered a state of affairs the place the information confirmed surprising or stunning insights? What’s your suggestion on coping with these situations?
Mr. Nirmal: One of many issues that we are likely to miss through the exploratory information evaluation (EDA) part is that- we would not be asking the proper inquiries to information. If we solely comply with the usual strategy of doing descriptive stats, uni- or multi variate evaluation, correlation warmth maps and so on, that are primary steps of EDA, chances are high we would miss discovering key insights.
One instance: The most typical course of to comply with after we encounter outliers in our information is to drop them, as a result of they’ll skew the distribution. Nevertheless, dropping them isn’t all the time a good suggestion, and it is dependent upon your undertaking. What if we’re doing an anomaly detection undertaking, then the outliers may be these anomalies that we’re looking for. On this case dropping from the coaching information isn’t a clever choice. It’s all the time higher to examine with the area specialists earlier than dropping any form of information, even the lacking information.
Recommendation to Change into Profitable Knowledge Scientist
AV: What recommendation would you give to somebody who needs to grow to be a profitable information scientist at a tech large like Microsoft?
Mr. Nirmal: My options usually are not solely restricted to Microsoft however apply on the whole to each trade and firm. If I’ve to summarize in few factors:
- Keep Hungry for Studying New Issues: The info science trade is all the time shifting at a quick tempo. Steady studying is essential on this subject.
- Construct your Community: Attend conferences, be a part of neighborhood channels in linkedin, contribute to neighborhood by writing articles in fashionable information science platforms like medium, or in the direction of information science. Networking helps lots.
- Give attention to Impactful Initiatives: The info scientist title can put you in lots of responsibilities- some doing information engineering work, some doing information analyst work. Regardless, I counsel you concentrate on excessive influence initiatives the place you can also make your contributions extra seen, and may be measured in tangible outcomes.
Conclusion
In closing, Mr. Nirmal’s success story serves as a shining instance of the unimaginable heights that may be achieved when expertise, alternative, and unwavering dedication converge. Microsoft’s Senior Knowledge Scientist has confirmed that the facility of information, when harnessed with brilliance and objective, has the potential to remodel industries, form the long run, and create a legacy that can endure for generations to return.
Lastly, I want to thank Analytics Vidhya for giving me this chance to share my expertise. To all my viewers, please be at liberty to attach with me on LinkedIn.