Many firms provide their purchasers promotional gives to shut offers, renew subscriptions, or buy providers. These incentives carry prices for the vendor when it comes to income or service that is being given to the client in trade for the acquisition. However when utilized appropriately, they might help make sure the transaction is dedicated and may even increase the scale of the acquisition. However not all accounts receiving an incentive provide will react in the identical manner. Utilized inappropriately, a promotional provide could don’t have any impression on the scale of velocity of a deal and/or could unnecessarily erode margins. It’s important for organizations proposing incentives to prospects to each anticipate the impact of the provide on the likelihood deal completion and perceive the impression it is going to have on the deal’s web profitability.
Optimizing Promotional Gives Can Result in Higher Outcomes
Take into account the fictional case of a software program firm that has been offering a number of varieties of promotional gives for a yr with out specific controls over how gross sales groups apply them to completely different proposals. When offers are introduced to completely different buyer accounts with differing desktop counts and ranges of income with out such controls, we would see random sprinkling of provide varieties throughout accounts of differing sizes (Determine 1). .
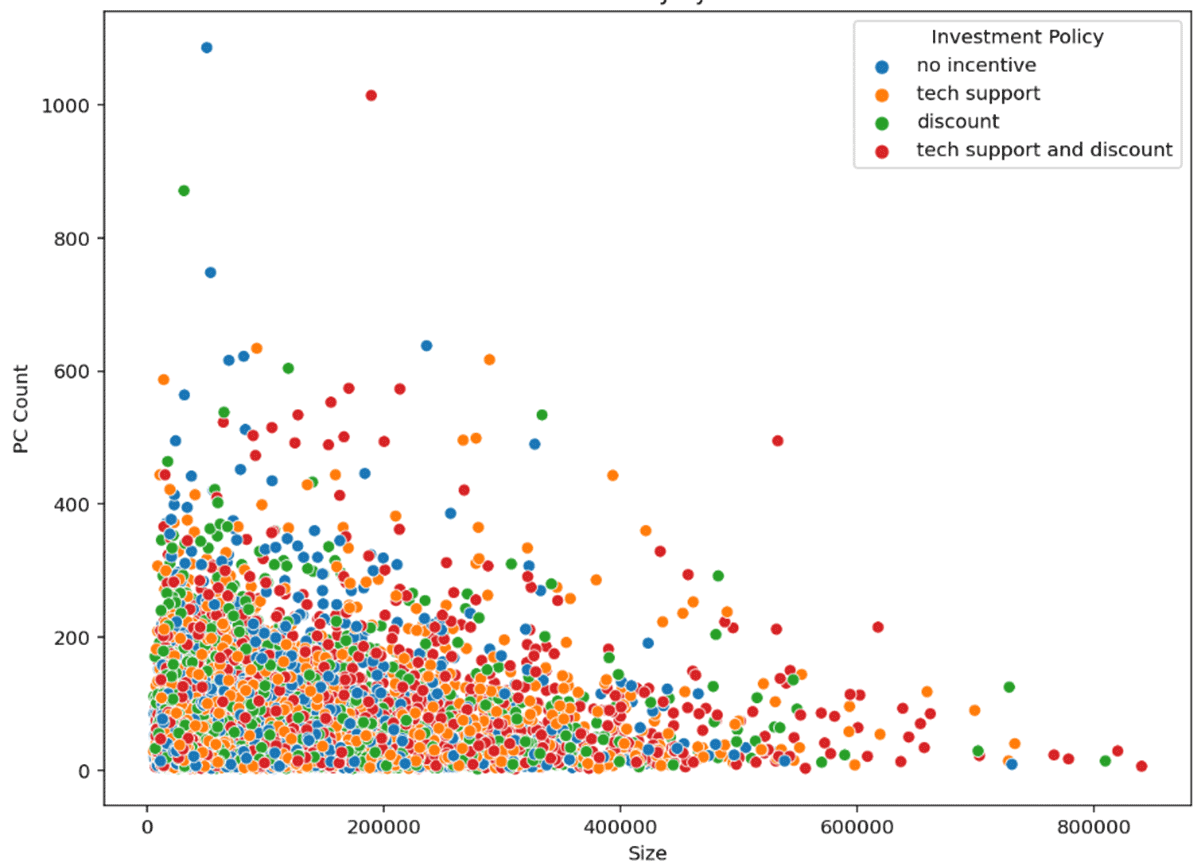
After we discover how these completely different gives have an effect on the likelihood of deal closure and margins for these offers, we will determine distinct combos of attributes that are inclined to result in optimum outcomes for the software program firm (Determine 2).
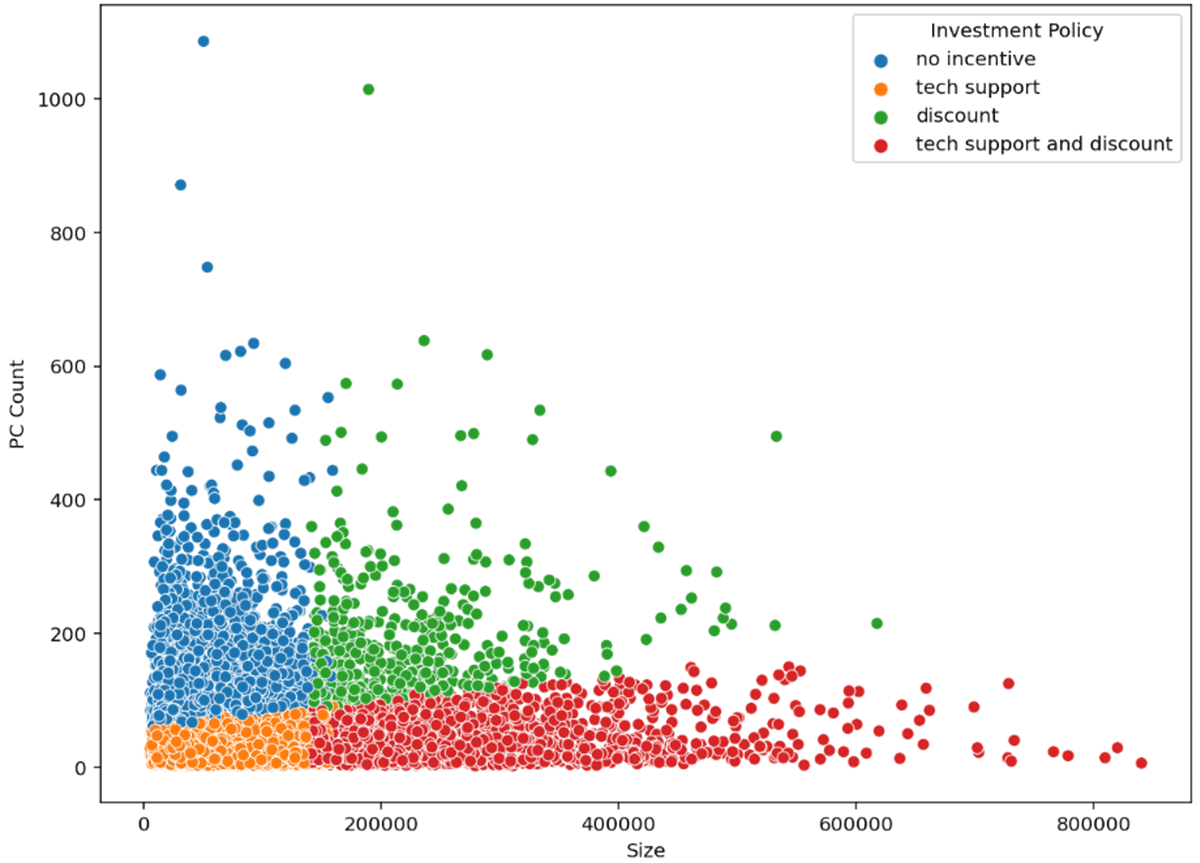
The outcomes of this work usually are not only a extra structured, programmatic manner of making use of promotional gives to offers, however as a substitute have actual implications for account margins. Take into account this comparability of various methods on the common margin for every account (Desk 1).
Coverage Description | Common Marginal Revenue per Account |
---|---|
Giving no promotional provide | $0.00 |
No coverage (No gross sales controls) | $312.85 |
All the time giving solely ‘tech assist’ | $1,816.19 |
All the time giving solely ‘low cost’ | -$1,684.62 |
All the time giving ‘tech assist’ and ‘low cost’ | $131.56 |
Giving optimized promotional provide | $4,961.88 |
Desk 1. The impression of various promotional provide insurance policies on common account margins
The desk reveals a marginal revenue enhance higher than $4,900 when utilizing the optimized method versus letting the gross sales crew resolve when to offer which promotional provide. The desk additionally signifies that recommending particular promotional gives in several account eventualities is best than some other naive method i.e. all the time given just one promotional provide, all the time given each promotional gives , and by no means giving a promotional provide
Causal Machine Studying Can Elicit the Drivers Behind These Outcomes
However how do we all know which gives to advocate in several eventualities in an effort to drive optimized outcomes? Utilizing new capabilities within the discipline of causal machine studying (Causal ML), we will mix an evaluation of probabilistic relationships within the knowledge with area information to ship fashions able to predicting which choices in a given state of affairs are prone to result in a desired consequence. It is some heady stuff to make certain, nevertheless it’s an space of energetic analysis that is permitting organizations to deal with powerful issues that beforehand have been past the attain of basic approaches.
To supply an introduction to Causal ML, we have collaborated with the crew behind the PyWhy ecosystem of open supply instruments for causal machine studying to reveal how these strategies might be utilized in a gross sales incentive optimization state of affairs. You possibly can obtain the technical notebooks loaded with explanatory element right here to start your journey on this house. We consider that when you’ve got been launched to how Causal ML approaches varied issues, you may start to see extra alternatives for its software in quite a lot of eventualities relative to your corporation.
Databricks Supplies a Platform for Causal Machine Studying
After you’ve got develop into acquainted with Causal ML and the way PyWhy supplies you the flexibility to implement options on this house, the pure subsequent query is, why Databricks? Databricks supplies a unified analytics platform for all knowledge and all modes of analytics, together with causal machine studying.
For a lot of prospects which have standardized on the Databricks Lakehouse Platform, implementing Causal ML options on Databricks is a pure evolution of their current predictive workflows. Knowledge scientists and knowledge engineers can collaborate on one platform, benefiting from the info processing horsepower offered by Databricks to organize the inputs for this work and leveraging native and third-party UIs for the evaluation of outputs with out the necessity for knowledge replication between environments.
As Databricks prospects success with the strategies, the mannequin deployment and internet hosting capabilities accessible with Databricks supplies a number of paths for the combination of those superior predictive capabilities with varied operational workflows. Briefly, Databricks removes the impediments to organizations pursuing their aims with their knowledge, permitting them to give attention to realizing the potential of the insights discovered inside them.
We want to give a particular thanks to the steering committee behind the PyWhy libraries for his or her assist and encouragement with this work.