In a groundbreaking transfer in the direction of addressing the approaching challenges of superhuman synthetic intelligence (AI), OpenAI has unveiled a novel analysis course – weak-to-strong generalization. This pioneering method goals to discover whether or not smaller AI fashions can successfully supervise and management bigger, extra subtle fashions, as outlined of their latest analysis paper on “Weak-to-Sturdy Generalization.”
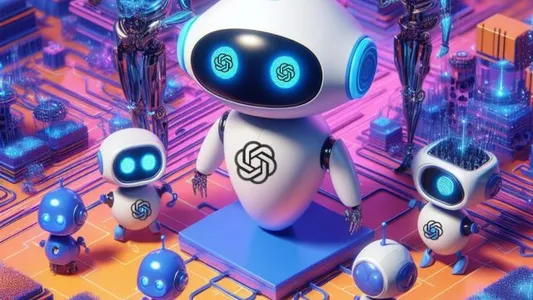
The Superalignment Drawback
As AI continues to advance quickly, the prospect of creating superintelligent programs inside the subsequent decade raises important issues. OpenAI’s Superalignment workforce acknowledges the urgent must navigate the challenges of aligning superhuman AI with human values, as mentioned of their complete analysis paper.
Present Alignment Strategies
Current alignment strategies, reminiscent of reinforcement studying from human suggestions (RLHF), closely depend on human supervision. Nonetheless, with the appearance of superhuman AI fashions, the inadequacy of people as “weak supervisors” turns into evident. The potential of AI programs producing huge quantities of novel and complicated code poses a big problem for conventional alignment strategies, as highlighted in OpenAI’s analysis.
The Empirical Setup
OpenAI proposes a compelling analogy to deal with the alignment problem: Can a smaller, much less succesful mannequin successfully supervise a bigger, extra succesful mannequin? The aim is to find out whether or not a strong AI mannequin can generalize in response to the weak supervisor’s intent, even when confronted with incomplete or flawed coaching labels, as detailed of their latest analysis publication.
Spectacular Outcomes and Limitations
OpenAI’s experimental outcomes, as outlined of their analysis paper, showcase a big enchancment in generalization. Utilizing a technique that encourages the bigger mannequin to be extra assured, even disagreeing with the weak supervisor when essential, OpenAI achieved efficiency ranges near GPT-3.5 utilizing a GPT-2-level mannequin. Regardless of being a proof of idea, this method demonstrates the potential for weak-to-strong generalization, as meticulously mentioned of their analysis findings.
Our Say
This revolutionary course by OpenAI opens doorways for the machine studying analysis neighborhood to delve into alignment challenges. Whereas the offered methodology has limitations, it marks an important step towards making empirical progress in aligning superhuman AI programs, as emphasised in OpenAI’s analysis paper. OpenAI’s dedication to open-sourcing code and offering grants for additional analysis emphasizes the urgency and significance of tackling alignment points as AI continues to advance.
Decoding the way forward for AI alignment is an thrilling alternative for researchers to contribute to the secure growth of superhuman AI, as explored in OpenAI’s latest analysis paper. Their method encourages collaboration and exploration, fostering a collective effort to make sure the accountable and helpful integration of superior AI applied sciences into our society.