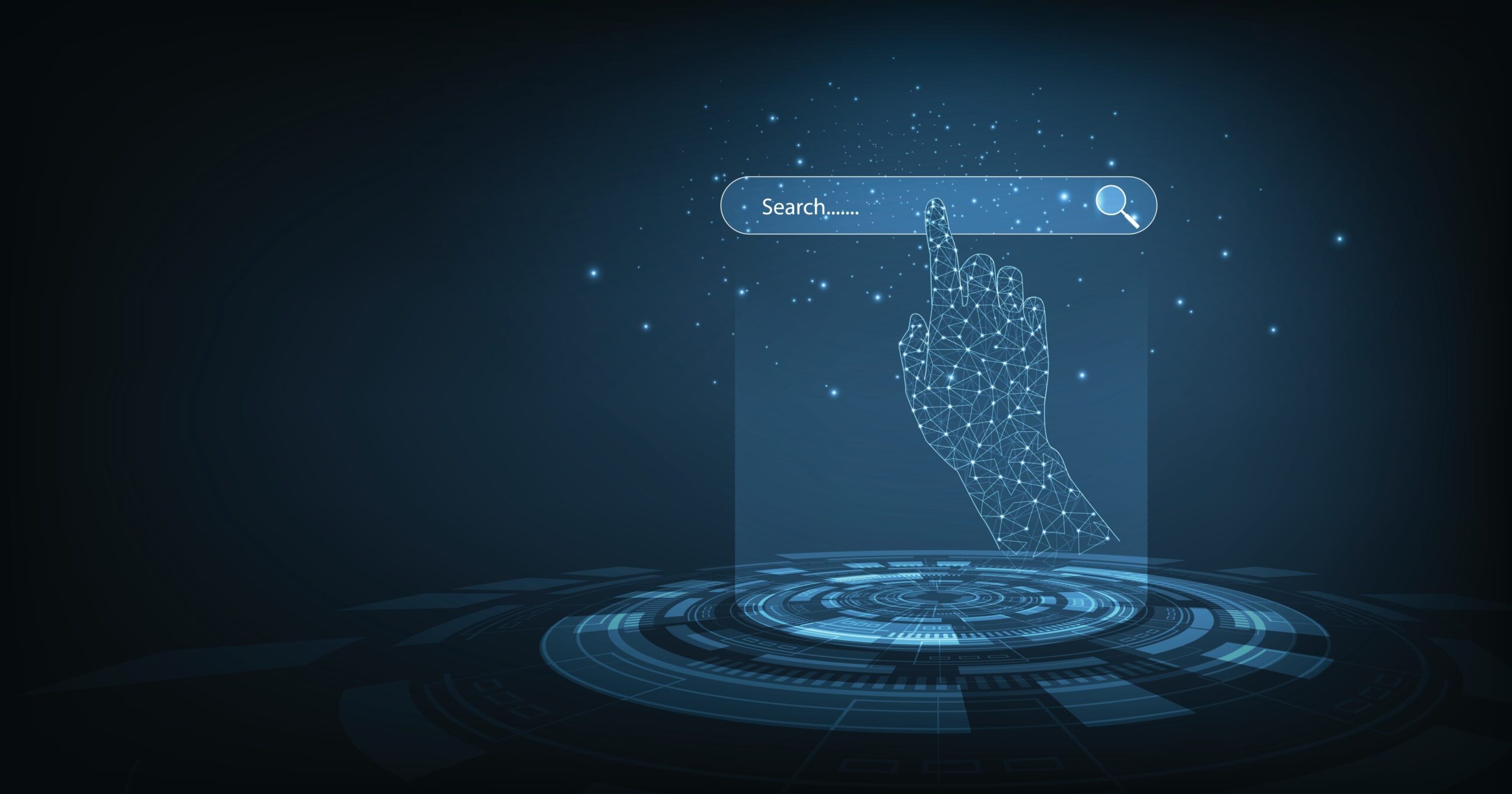
(Chor muang/Shutterstock)
Actual-time analytics have solidified their place as a cornerstone throughout quite a few industries. Individually, the attract of generative AI has captured widespread consideration, promising revolutionary options and unprecedented insights in fields starting from leisure to healthcare. The convergence of real-time analytics utilizing generative AI strategies presents a compelling synergy. It equips organizations to uncover deeply hidden insights in instances when the chance is perishable.
DJ Patil, former Chief Knowledge Scientist of the US, and former Chief Scientist at LinkedIn, says that totally harnessing the potential of generative AI will necessitate the event of capabilities centered on fast knowledge processing.
“Many of the stuff we see round LLMs immediately is low-speed knowledge; it’s very static, and it hasn’t been up to date,” Patil says. “That’s one thing I believe we’re going to see develop over the subsequent 24 months.”
One of many revolutionary applied sciences on the coronary heart of generative AI are vector databases. Consider these as organized collections of knowledge that take sample matching to new heights. A vector embedding is a manner of organizing knowledge that makes it simpler to search out similarities and relationships between totally different items of knowledge. To date, vector databases have been restricted by stale, historic knowledge. Customers of ChatGPT are conversant in the truth that it’s blind to any info created after September 2021.
With the intention to totally respect the immense potential of real-time generative AI, it requires that we shift our perspective away from the myopic notion that generative AI is confined to artistic domains like music, visible arts, and prose. Whereas these creative purposes have undoubtedly showcased the know-how’s capabilities, the scope of generative AI extends far past these realms. It holds the facility to optimize numerous sectors the place fast-moving knowledge from sensors and machines are essential for decision-making.
How will it change the best way companies function? In monetary companies, I believe we’ll see real-time vector search revolutionize fraud detection and danger evaluation. By encoding historic transaction knowledge and buyer profiles as vectors, you possibly can quickly match incoming transactions in opposition to recognized patterns of fraudulent habits. This may allow prompt identification of suspicious actions, resulting in faster response instances and lowered monetary losses.
Moreover, danger evaluation fashions will leverage real-time vector embeddings to offer up-to-the-moment evaluations of market circumstances, optimizing funding choices. For instance, think about funding banking’s use of VWAP, quick for volume-weighted common worth, which serves as a technical evaluation instrument revealing the connection between an asset’s worth and its whole commerce quantity. It affords merchants and buyers a way to evaluate the common worth at which a inventory has been traded throughout a specified timeframe.
Consider VWAP as a possible vector embedding, of which there’s one for every inventory, throughout every buying and selling desk, throughout a number of home windows in time, leading to 1000’s of vector embeddings created every day. Now think about that VWAP is however one in all dozens of economic metrics used to make a purchase or promote willpower in real-time, necessitating extra vector embeddings. If each inventory maintained quite a few repeatedly up to date vector embeddings to replicate market circumstances, it will unveil unprecedented patterns and alternatives within the monetary panorama. As an example, “present me the highest three shares poised to interrupt out to the upside within the subsequent 5 days.”
Logistics is one other space ripe for change by coupling generative AI with the wealth of sensor readings from autos, containers, warehouses, conveyor techniques, packaging, and extra. Via ongoing evaluation in dynamic circumstances, companies can optimize route planning, scale back supply instances, decrease spoilage, and decrease stock holding prices. It gained’t solely streamline logistics however may also equip organizations with the agility required to reply promptly to unexpected disruptions.
Actual-time vector search holds immense potential in protection purposes, notably for menace looking and intelligence evaluation. On this context, vector embeddings may characterize options equivalent to radar signatures, satellite tv for pc imagery, or intercepted communication patterns. Actual-time vector search techniques will swiftly evaluate incoming knowledge to a complete database of recognized threats and anomalies. It will allow army and safety personnel to quickly establish potential threats, equivalent to new aerial spy autos or suspicious troop actions and make knowledgeable choices accordingly.
Any business that’s already benefiting from real-time analytics will discover this breakthrough in sample matching will take present use instances to the subsequent stage. In retail, it is going to make recommender techniques extra correct by matching buyer preferences to out there merchandise. Within the automotive sector, it is going to improve superior driver help techniques by means of real-time recognition of objects and highway circumstances. In manufacturing, it is going to optimize high quality management by quickly figuring out defects in manufacturing strains. Within the vitality sector, it is going to streamline grid administration and predictive upkeep for improved effectivity. In utilities, it is going to bolster infrastructure monitoring, lowering downtime and guaranteeing dependable service supply.
To help these use instances, the important thing know-how shift is from batch-oriented vector databases to real-time vector databases. We’re seeing improvements like NVIDIA’s framework for GPU vector search that’s paving the best way for real-time insights based mostly on vector embeddings.
In regards to the creator: Chad Meley is the Chief Advertising Officer for Kinetica, a supplier of GPU-accelerated analytics options. Chad’s has greater than 20 years of expertise as a frontrunner in SaaS, huge knowledge, superior analytics, the place he has offered data-driven advertising, technique and planning for early-stage software program corporations and huge, established leaders alike. Previous to becoming a member of Kinetica, Chad was VP of Product Advertising at Teradata. Chad has additionally held quite a lot of management roles centered on knowledge and analytics with Digital Arts, Dell and FedEx. Chad holds a doctorate from the College of Florida the place his dissertation was on Utilized Synthetic Intelligence, an MBA from the Rawls School of Enterprise at Texas Tech College and a B.A. in Economics from the College of Texas.
Associated Gadgets:
Retool’s State of AI Report Highlights the Rise of Vector Databases
Vector Databases Emerge to Fill Important Function in AI