Organizations wish to ship extra enterprise worth from their AI investments, a scorching subject at Huge Knowledge & AI World Asia. On the well-attended information science occasion, a DataRobot buyer panel highlighted innovation with AI that challenges the established order. A packed keynote session confirmed how repeatable workflows and versatile know-how get extra fashions into manufacturing. Our in-booth theater attracted a crowd in Singapore with sensible workshops, together with Utilizing AI & Time Collection Fashions to Enhance Demand Forecasting and a technical demonstration of the DataRobot AI Cloud platform. Â
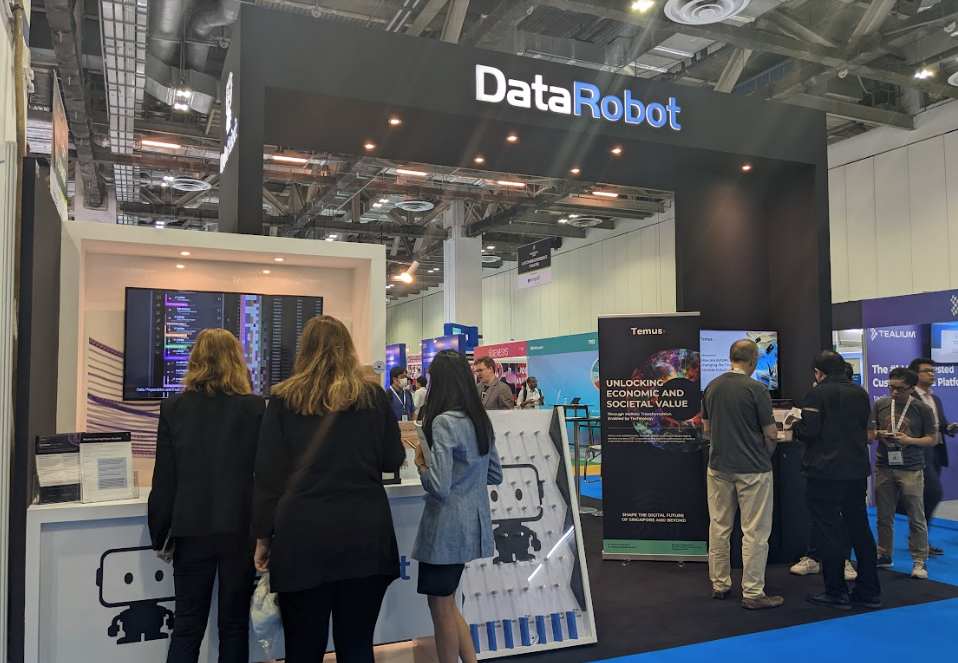
Automate with Fast Iteration to Get to Scale and Compliance
Monetary Companies leaders perceive the significance of pace and security. On the occasion, a monetary companies panel dialogue shared why iteration and experimentation are essential in an AI-driven information science surroundings.Â
Sara Venturina, VP Head of Knowledge from GCash, the Philippines’ main e-wallet, and Trevor Laight, Chief Threat Officer from CIMB, a number one ASEAN common financial institution, hosted a dialogue panel with Jay Schuren, DataRobot Chief Buyer Officer.Â
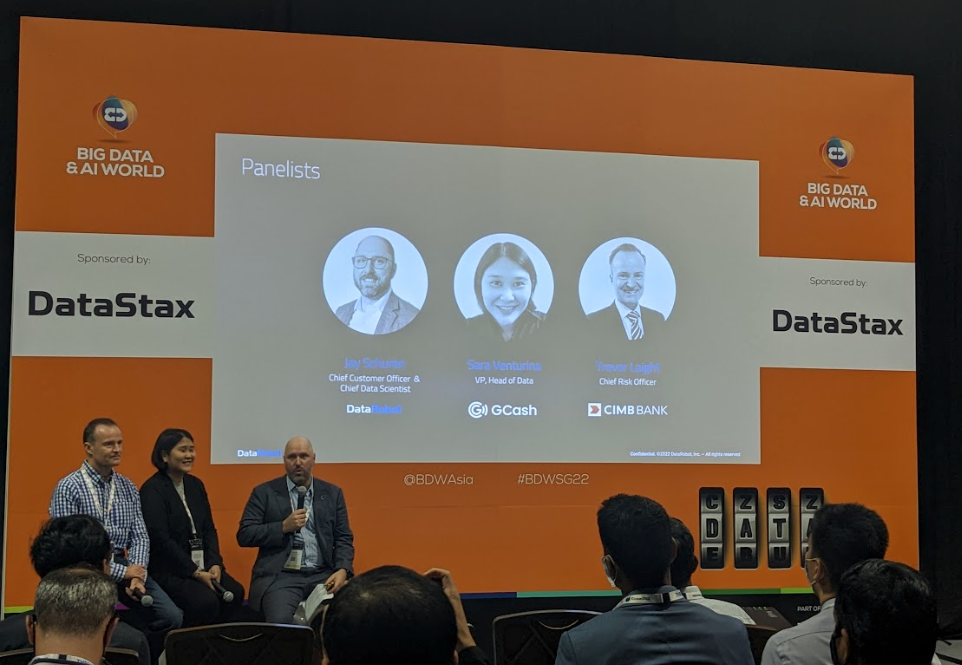
The panel dialogue centered on Boyd’s Regulation of Iteration—a idea from dogfighting (army aviation technique) which believes that the pace of iteration beats the standard of iteration.Â
Trevor defined how this mindset of speedy iteration has been essential to maintain tempo with the evolving wants of the enterprise. He strengthened that the power to make use of automation inside the experimentation and iteration phases has allowed CIMB, to proceed to scale—even within the midst of distinctive information challenges and a fancy regulatory surroundings.
With DataRobot AI Cloud, Trevor is ready to mix his folks’s finest experience with the facility of automation to drive repeatable experimentation at scale and be certain that the very best mannequin makes it into manufacturing.
Sara added that driving digital transformation was not only a know-how initiative—however reasonably an all-encompassing change administration train. Whereas GCash has been rising exponentially as a disruptor within the monetary market, the significance of having the ability to deliver everybody alongside on the journey—even non-technical stakeholders—is essential. Â
With DataRobot, Sara has the power to elucidate the fashions that her Knowledge Science staff is creating and may robotically generate the required compliance documentation. This enables GCash to keep up the tempo of innovation and iteration with out exposing the enterprise to vital threat.
Closing the Worth Hole: Lowering AI Cycle Time
What occurs while you attempt to remedy complicated issues in silos—with out the alignment of essential stakeholders? You spawn the dreaded AI worth creation hole. Ted Kwartler, VP of Trusted AI, DataRobot, shared a keynote handle that put this creation hole below the microscope—and confirmed how AI governance can result in sooner worth creation.
Knowledge scientists in lots of organizations are below undue stress to slender this worth hole. Ted defined that—by working in silos—most companies are getting fashions from their Knowledge Science staff that then should be rewritten by IT earlier than lastly transferring into manufacturing. These fashions don’t permit for monitoring over time, have little or no documentation, and don’t meet the basic wants of the enterprise.
Closing the worth hole and decreasing the general AI cycle time means addressing the person wants of every stakeholder group inside the machine studying lifecycle. Ted highlighted 4 key stakeholder wants:
- AI Innovators have a strategic lens and are trying on the general ROI of the AI undertaking whereas assessing essential components like belief and threatÂ
- AI Creators look via a technical lens and deal with defining and constructing the correct mannequinÂ
- AI Implementers deal with deploying, sustaining, and monitoring the mannequin over time and are accountable for general system well being
- AI Customers be certain that a mannequin suits with organizational values, compliance, authorized, and regulatory necessities
With a view to meet these wants, Ted enumerated the usual governance questions that organizations want to handle:
- Is the code straightforward to learn and perceive?
- Is the mannequin explainable, traceable, and auditable?
- Is the mannequin reproducible?
- Can we be assured that it’ll meet regulatory necessities?
Explainability spans throughout your complete DataRobot platform to assist customers at every step. World rationalization strategies permit stakeholders to know the habits of fashions and the way options have an effect on them. Native explanations present row-level explanations for why a mannequin made a prediction. Prediction explanations share which options and values contributed to a person prediction and their affect.Â
DataRobot affords automated documentation that helps pace the documentation course of for fashions with deployment reviews and compliance reviews that define mannequin methodologies and efficiency.Â
Simplify Your Tech Stack with Interoperable, Versatile InstrumentsÂ
At Huge Knowledge & AI Asia, DataRobot groups additionally mentioned how flexibility and interoperability within the machine studying know-how stack will help derive worth from AI initiatives. Machine Studying stacks are generally fragmented and laborious to handle throughout departments, creating complexity and value that may inhibit scale and decelerate progress. Organizations which might be simplifying their stacks—with a bias in direction of instruments which might be versatile throughout the storage, improvement, and consumption layers—are higher positioned to seize worth.
DataRobot affords flexibility and interoperability with the broadest multi-cloud and hybrid deployment choices, permitting groups to leverage the infrastructure they have already got in place. Broad ecosystem integrations additionally allow groups to work with the information the place it resides, minimizing complexity and permitting for simple consumption.Â
Study The way to Speed up Enterprise Outcomes with DataRobot AI Cloud  Â
Study extra concerning the DataRobot AI Cloud platform and the power to speed up experimentation and manufacturing timelines. Discover the DataRobot platform in the present day.
In regards to the writer
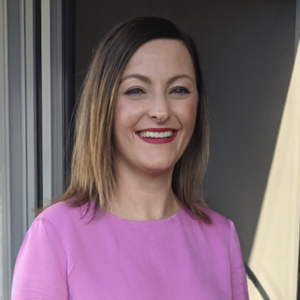
Director, Demand Planning, Artistic & APAC Advertising at DataRobot
Brook leads APAC Advertising and Demand Planning for DataRobot. Having spent the final decade of her profession working with among the largest and quickest rising know-how firms, she believes that the majority efficient advertising and marketing is developed from a strong buyer perception, knowledgeable by significant information and formed by sensible inventive pondering. Brook is passionate concerning the potential for AI to drive constructive change.