Media In our on a regular basis life, it has develop into more and more arduous to keep away from listening to the phrases ‘Synthetic Intelligence (AI)’ and ‘Machine Studying (ML)’ whether or not in trade or academia. These applied sciences have entered our every day lives and are remodeling most sectors of the economic system, constructing new spheres of information and observe, and ushering a brand new period in human historical past. But whilst these types of quickly evolving intelligence develop into extra seen inside and outdoors the academy, their imprecise definitions, vagueness about their modalities and scope of purposes impedes their full understanding. This text goals to make clear these new applied sciences, distinguish them from one another, and description their sweeping implications.
1. AI/ML on the planet of IoT
Synthetic Intelligence (AI), Machine Studying (ML) and the Web of Issues (IoT) are intricately linked and collectively symbolize a strong triad, ushering in a brand new wave of innovation. The trio is enabling a brand new technology of good, self-adjusting and self-optimising autonomous merchandise and machines, which in flip are disrupting and remodeling each sector from manufacturing to well being care. The hyperlink between AI and ML and IoT is a pure one:
-
Knowledge-driven intelligence:
The turbines of this information are sensors and good units embedded in on a regular basis objects, in contexts as various as visitors networks or kitchen home equipment. It’s the energy and prowess of AI and ML that present the computational intelligence to course of, remodel and analyse the information, and switch it into actionable data. IoT kinds the data-capturing layer, whereas AI and ML symbolize the evaluation engine that constitutes the computational mind.
In trade, IoT units monitor sensors of kit and equipment. ML algorithms can determine hyperlinks between its present information and historic information, after which predict machine or tools failure, upkeep wants and different points. Your entire course of is steady, and the ML algorithm can predict machine circumstances primarily based on real-time information from IoT units. For example, if oil ranges are low or there was extreme vibration, the programs might predict a possible machine breakdown. On this manner, predictive upkeep can minimise downtime and decrease materials prices with out considerably rising labour prices.
-
Enhanced consumer expertise and personalisation:
Examples of those shopper purposes will be present in IoT units that accumulate details about customers’ interactions and preferences. For example, by analysing how you utilize a wise residence, AI might management your lighting and temperature primarily based in your behaviour, with machine studying algorithms bettering the predictive efforts over time should you proceed to make use of it. Health trackers may also use ML algorithms to personalise well being suggestions.
-
Autonomous decision-making:
Utilizing AI and ML, IoT units can start to take autonomous choices primarily based on real-time information. Autonomous automobiles (an ecosystem of IoT units), for instance, use ML to know sensor information and resolve which driving actions to take moment-by-moment on the street. In our houses and places of work, vitality grids use AI to stability grid hundreds and intelligently optimise vitality distribution primarily based on IoT information offered in actual time.
Safety and cyber assaults can creep into IoT networks. AI and ML can work like safety radar and detect anomalies within the state of IoT networks or within the information generated by IoT units to inform whether or not an assault is going on or about to occur. AI-powered safety can due to this fact make IoT safer – these programs can all the time be taught from the information coming from the networks and replace the measures to undertake.
In enterprise and manufacturing, IoTs enter a variety of variables and parameters that are analysed by ML algorithms to optimise operations by reducing down on the quantity of waste and bettering effectivity. AI on the identical time can be utilized to automate extra complicated decision-making processes, and on this technique to optimise, in actual time, the operation parameters.
In brief, AI and ML are indispensable to IoT, and a intelligent system will combine all three as an clever ecosystem of studying, adapting, and deciding: an IoT driver and accelerator of future improvements, itself helped alongside by good regulation.
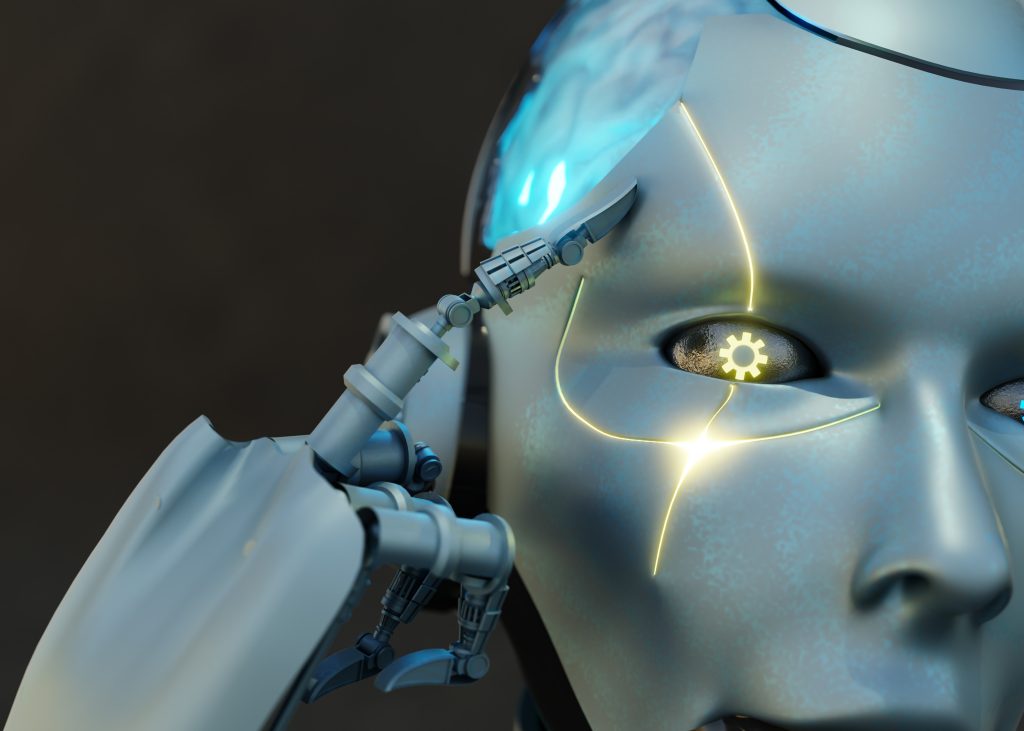
2. Decoding AI and Machine Studying: A comparative overview
Synthetic Intelligence (AI)
Synthetic Intelligence is a subject – or we might even say self-discipline – of pc science that goals to create programs able to performing duties which can be generally considered requiring human intelligence. A few of its important marks revolve round the usage of ideas corresponding to intelligence and studying, by which one correlates the AI’s task-performing functionality with the human’s college of cognition. Examples of such duties embody the understanding of pure language – which could resonate with the human capacity to talk; the sample recognition – intently associated to the human college of notion; and the inherently comparable capacity to resolve complicated issues that contain unforeseeable difficulties and inexorable vagueness and uncertainty as to their options – like puzzles drawn from the actual world for the intellectually curious human. It’s extensively held that, whereas chatbots are extra narrowly goal-oriented, AIs contain the flexibility of computer systems to carry out any of the abovementioned duties in a manner that will trigger us to say the pc is ‘good’. That is what is usually referred to as ‘mental mimicry’ or ‘mimesis’ of the human intelligence – in brief, to be taught from expertise and ‘act cleverly’.
Machine Studying (ML)
Machine Studying considerations a very lively space of Synthetic Intelligence (AI) that tries to codify the flexibility of computer systems to be taught, make selections or predictions primarily based on information, bypassing the necessity for human enter or steerage. Algorithms are skilled on beforehand gathered information units till they perceive the underlying patterns of that information, make knowledgeable selections primarily based on what they’ve realized, and are able to persevering with to incrementally enhance their predictive capability autonomously going ahead. ML’s goal is to develop programmes which can be able to exploiting information as a way to develop into higher, extra adaptable at studying on their very own, with out intervention, process by process.
Key Variations:
AI is for constructing an clever pc that solves the issues within the analogous manner as a human does, whereas ML permits for a robotic to be taught from the information in order to attract an correct forecast.
Performance: the machine makes use of a pre-written rulebook (typically the system flexes and ‘tweaks’ guidelines primarily based on outcomes) versus the ML system follows a pattern-cloud of anticipated inputs that results in a solution.
3. What they bring about to the desk: Capabilities and purposes
AI’s contributions:
Synthetic intelligence is kind of good at automating routine duties – whether or not these are simple jobs corresponding to information entry, or the hidden processes that feed these choices, it maximises effectivity and productiveness.
Due to cognitive companies (for language understanding, speech, and imaginative and prescient), the pc is provided with a wider vary of interplay with people.
AI programs can attain significant conclusions concerning the current by evaluating and contrasting previous and current information, making knowledgeable connections and syntheses of reams of enter.
ML’s contributions:
ML fashions are wonderful at prediction and forecasting tendencies and behaviours from previous information, and that is the place they are often utilized, like within the monetary, medical and advertising and marketing sectors.
Probably the most profitable ML purposes is studying to recognise buried patterns in information, corresponding to anomalous exercise in cybersecurity or tell-tale indicators of illness in diagnostic medication.
ML produces customized consumer experiences relying on how a person consumer has interacted with the service previously, and is likely to be used to enhance companies for e-commerce, leisure and rather more.

4. The synergistic relationship: How AI and ML complement one another
That relationship can be mutually supportive, with the science in a single feeding again to enhance and inform the opposite nascent science, and the ensuing programs rising extra succesful and cognitively highly effective over time. AI is the organiser: the sector of synthetic intelligence defines the general objectives and architectures for constructing machines that, in precept, can exhibit facets of human intelligence. ML is the toolkit: the sector of machine studying provides the strategies and methods that permit these machines to be taught issues from information, get higher with observe, and make choices.
- Enhanced studying capabilities: So tuning into real-world ‘vibrations’ of humanity is essential. AI programs are meant to learn by human intelligence and ML permits machines to be taught from expertise, in the best way that people do. If statistical studying bridges machines and people, then ML has some promise – the data-driven programs should learn to ‘recalibrate’ (as people do) when confronted with new examples of ‘human’ behaviour (for eg, driving, interacting with different people, and so forth).
- Knowledge-driven determination making: Being ‘good’ in AI means ‘being a very good decision-maker’. ML is (most likely) a null speculation for a way finest to make AI quick at making choices by giving it the instruments to analyse a variety of information about regardless of the AI objects are doing every second, determine what the patterns are in that information, after which (makes a prediction) use evaluation and pattern-recognition to make the subsequent determination.
- Predictive energy and personalisation: In lots of different methods, ML is an efficient software to make potential what AIs are designed to realize for customers: personalised expertise and consequence prediction. ML is nice in delivering a personalised expertise to a consumer of an e-commerce web site, a video streaming service or a customer-service platform as a result of it takes information factors about what the consumer has achieved previously and predicts what that consumer will finally do.
- Autonomous enchancment: A elementary facet of the idea of AI is the flexibility to design an autonomous system. ML takes this a step additional, since programs usually are not simply being designed to function autonomously, however to optimise efficiency autonomously (for instance, by studying from the information obtained after beginning up). Within the case of a system corresponding to an autonomous automobile that should ‘be taught’ the best way to cope with an unknown surroundings, such an enchancment loop is crucial. Advanced.
- Drawback-solving: Aupiter AI seeks to deal with the intractable within the aforementioned computational house of real-world issues, the place all sensible options seem like hopelessly sophisticated, and the apparent, simple avenues run aground. ML compounds the complexity by delivering a paradigm primarily based on mixing a number of modalities (eg, units of algorithms corresponding to neural nets) to match real-world complexity and harness excessive complexity, largely unstructured information largely out there in the actual world.
Put the 2 collectively, and you’ve got an exponentially accelerating technological ecosystem – by which ML’s capacity to construct ‘inductive’ fashions, and be taught to be taught by iterative growth from information, will be allied with AI’s much more formidable agenda, of modelling human intelligence to create ever extra generalist ‘generative’ programs that may grasp all kinds of complicated duties, blast by way of innovation boundaries, and turbocharge whole industries.
5. Challenges and moral issues
With nearly each fast-advancing and doubtlessly disruptive applied sciences for AI and of machine studying (ML), we quickly discover that considerations about how the know-how would possibly change the world evolve nearly as shortly because the rising and quickly advancing applied sciences themselves. It’s an unprecedented problem: as a result of AI and ML programs want quantity of knowledge to function successfully, we generate worries about information safety and privateness. The broader moral considerations embody the problems of bias and equity within the design of AI (ie, algorithms would possibly produce biased outcomes as a result of they’ve been skilled earlier on biased information) and that intentional determination processes applied by algorithmic are extra interpretable and open than human ones – particularly in instructional, medical and legal justice situations, the place transparency will be as necessary as a call itself. There shall be jobs eradicated within the automation course of, a scenario requiring much-needed workforce administration and worker retraining methods – and so forth. In reality, this may be paraphrased to: THE BIG WORRIES:
At the forefront of this work are rising calls to enunciate ideas and impose requirements for the design and deployment of AI and ML applied sciences. That is going to require a large-scale partnership throughout firms, policymakers and different stakeholders to make sure that AI and ML applied sciences are developed and deployed securely, pretty, transparently and for the general public good.
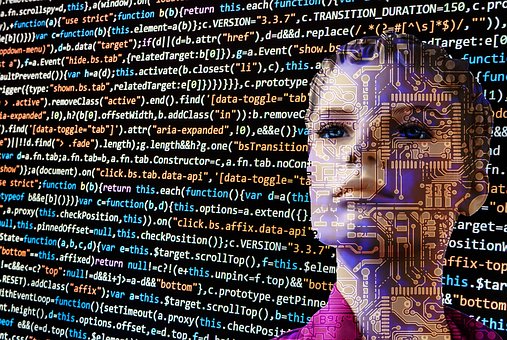
6. The long run outlook: Infinite prospects
As soon as extra, on the cusp of the subsequent know-how revolution – in AI and ML – the identical applies: medication will remodel as sufferers are prescribed therapies primarily based on tomographic scanning of their DNA; our city lifeworlds shall be recast in AI-driven ML cities deployed all through our infrastructure.
Taken collectively, AI and ML are enabling a future, more and more seamless and invisible, by which know-how underpins a lot of our actuality. Understanding what separates them, what they will accomplish and the place they may proceed to hit partitions is one thing that organisations, coverage makers and the overall inhabitants alike shall be effectively served to know within the years to return. With these applied sciences nonetheless evolving, fully new worlds will emerge, others fall away, and the world round us will proceed altering by way of eyes that can’t but start to see. The AI revolution is just simply starting. The chances are as limitless as our imaginations will permit.
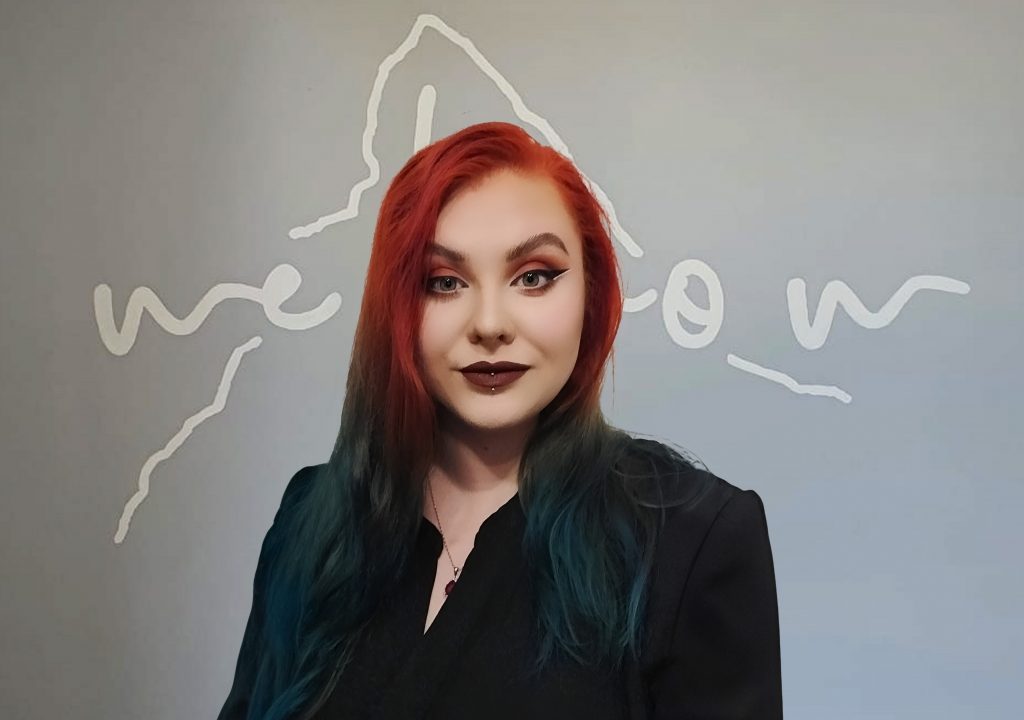
Article by Magda Dąbrowska, a technical author at WeKnow Media
Touch upon this text under or by way of Twitter: @IoTNow_