In at present’s digital panorama, the fusion of machine studying (ML) capabilities with net functions have ushered in a brand new period of innovation and performance. Amongst these sturdy frameworks, Ruby on Rails stands out for its effectivity and ease of use, making it a most popular alternative for a lot of builders. When mixed with the facility of machine studying, it unlocks new potentials in predictive analytics, personalization, and automatic decision-making.
Constructing upon the muse of integrating machine studying capabilities with Ruby on Rails in net functions, the position of builders on this dynamic panorama is essential and multifaceted. To develop options with ML capabilities, the builders at House-O Applied sciences, Wipro, Accenture, and Google carry their very own distinctive strengths to construct RoR options with ML.
The experience and dedication of builders at House-O Applied sciences in harnessing the potential of machine studying algorithms inside net growth have propelled industries towards a future the place clever, adaptive, and user-centric functions thrive. Let’s perceive the evolution and advantages of ML in net functions.
The Evolution of Internet Purposes and Machine Studying
Conventional net functions had been primarily static, providing primary functionalities and restricted interactivity. Nevertheless, with the appearance of dynamic net applied sciences and the exponential development of information, the panorama has shifted. Machine studying algorithms, powered by giant datasets, have remodeled how net functions function.
ML capabilities have enabled these functions to investigate patterns, predict consumer conduct, and adapt in real-time, resulting in a large number of advantages throughout varied industries.
High Advantages of Constructing Subsequent-gen Internet Apps with ML Capabilities
Improved Consumer Engagement
Enhancing consumer engagement lies on the core of ML-enabled net functions. Via dynamic interfaces that evolve with consumer interactions, these apps create an immersive and fascinating expertise. As an illustration, social media platforms leverage machine studying to prioritize content material on a consumer’s feed, retaining them engaged by showcasing related posts. Moreover, sensible notifications triggered by ML algorithms, akin to reminders or suggestions, not solely maintain customers knowledgeable but additionally entice them to remain linked and work together with the app.
- Dynamic Interactions: ML-powered net apps can adapt in actual time primarily based on consumer interactions, providing a dynamic and responsive consumer interface.
- Good Notifications: Customized and well timed notifications or strategies improve consumer engagement, retaining them knowledgeable or engaged with the app’s choices.
Environment friendly Information Processing
The incorporation of ML capabilities streamlines information dealing with and evaluation inside net functions. By using machine studying algorithms, these functions can course of huge quantities of information swiftly, extracting significant insights in actual time. This agility in information processing facilitates faster decision-making and adaptation to altering consumer wants. Furthermore, automation of duties, akin to information entry or categorization, not solely reduces human effort but additionally minimizes errors, making certain the accuracy and reliability of data.
- Sooner Insights: ML accelerates information evaluation, offering quicker insights into consumer conduct or traits.
- Job Automation: Automation of repetitive duties streamlines operations, decreasing workload and growing effectivity.
Superior Safety Measures
Integrating machine studying enhances the safety of net functions by enabling clever risk detection and prevention mechanisms. ML algorithms analyze patterns in consumer conduct, figuring out potential safety dangers, and promptly responding to mitigate them. This proactive strategy considerably strengthens the applying’s defenses in opposition to cyber threats. Moreover, real-time anomaly detection permits for swift motion in opposition to any suspicious actions, making certain information integrity and consumer confidentiality.
- Menace Detection: ML-based safety programs determine and reply to potential threats in real-time.
- Anomaly Detection: Detecting irregular patterns aids in stopping safety breaches earlier than they happen.
Optimized Useful resource Allocation
Machine studying integration empowers net functions to foretell useful resource wants primarily based on consumer demand patterns. By analyzing historic utilization information, these functions allocate assets optimally, making certain easy operations even throughout peak utilization. This predictive allocation not solely enhances efficiency but additionally minimizes wastage, resulting in cost-effective useful resource administration. Moreover, the scalability achieved by way of environment friendly useful resource utilization allows functions to seamlessly adapt to various consumer hundreds with out compromising efficiency.
- Predictive Allocation: ML predicts useful resource calls for, optimizing allocation for higher efficiency.
- Scalability: Environment friendly useful resource utilization allows scalable efficiency primarily based on consumer load.
Smoother Consumer Interface
The mixing of machine studying into net apps revolutionizes consumer interfaces by introducing pure language processing capabilities. Customers can work together with functions by way of voice instructions or text-based queries, simplifying the consumer expertise. Moreover, ML-driven UI enhancements guarantee intuitive navigation and usefulness, adapting to consumer preferences and conduct. These enhancements lead to a extra seamless and user-friendly interface, enhancing general consumer satisfaction and retention.
- Pure Language Processing (NLP): Implementing NLP enhances consumer interactions by way of voice instructions or text-based queries.
- UI Enhancements: ML-driven enhancements streamline navigation and usefulness for a extra intuitive expertise.
Dynamic Content material Era
Machine studying facilitates the creation of dynamic and personalised content material inside net functions. By analyzing consumer preferences and conduct, ML algorithms generate suggestions tailor-made to every consumer’s pursuits. For instance, streaming platforms make the most of these capabilities to recommend films or reveals primarily based on viewing historical past, growing consumer engagement. Furthermore, the flexibility to customise content material creation primarily based on particular person consumer conduct ensures a extra related and fascinating expertise, fostering a deeper connection between customers and the applying.
- AI-generated Suggestions: ML algorithms create personalised content material strategies primarily based on consumer preferences.
- Personalized Content material: Tailoring content material creation to swimsuit particular person consumer pursuits and conduct.
Actual-time Insights and Resolution Making
The incorporation of machine studying capabilities empowers net functions to derive real-time insights from information. By processing data swiftly, these functions present instant suggestions and evaluation, enabling agile decision-making. Moreover, the continual studying side of ML algorithms ensures that the system adapts and improves its decision-making primarily based on new information, fostering a extra responsive and correct decision-making course of.
- On the spot Evaluation: ML allows fast information evaluation for instant insights into consumer conduct or traits.
- Steady Studying: Algorithms adapt and be taught in real-time, bettering decision-making over time.
Automation and Course of Optimization
Machine studying integration results in automation and optimization of processes inside net functions. By automating repetitive duties, ML frees up human assets, permitting for a extra concentrate on complicated duties that require human intervention. Furthermore, ML-driven optimization of processes minimizes errors and inefficiencies, leading to streamlined operations and improved general efficiency. These developments not solely increase productiveness but additionally improve the reliability and consistency of processes inside the utility.
The mixing of machine studying capabilities into next-gen net functions revolutionizes functionalities, consumer experiences, and operational efficiencies, finally redefining the potential of web-based options throughout numerous industries.
- Workflow Automation: ML automates repetitive duties, boosting productiveness and effectivity.
- Optimized Processes: Algorithms optimize processes, minimizing errors and streamlining operations.
Adaptive Buyer Assist
Machine studying integration enhances buyer assist inside net functions by way of clever chatbots and AI assistants. These instruments leverage pure language processing and historic information to offer personalised and responsive customer support. By analyzing consumer queries and conduct, ML-powered chatbots supply tailor-made options, resolving points effectively. Moreover, predictive subject decision capabilities preemptively determine and deal with potential points, minimizing consumer frustration and making certain a smoother customer support expertise.
- Chatbots and AI Assistants: ML-powered chatbots supply personalised and environment friendly buyer assist.
- Predictive Challenge Decision: Algorithms anticipate and resolve potential points earlier than they escalate.
Steady Studying and Enchancment
The mixing of machine studying fosters steady studying and enchancment inside net functions. ML algorithms regularly refine their fashions primarily based on new information, bettering accuracy and efficiency over time. Furthermore, consumer suggestions performs a pivotal position on this course of. By integrating consumer suggestions into the educational loop, functions can adapt and evolve primarily based on consumer experiences, making certain ongoing enhancements that align with consumer preferences and expectations.
- Algorithmic Refinement: ML algorithms constantly be taught and refine their fashions for higher efficiency.
- Suggestions Utilization: Integration of consumer suggestions for algorithmic enchancment and adaptation.
Environment friendly Content material Moderation
Machine studying capabilities facilitate environment friendly content material moderation inside net functions. Via automated filtering mechanisms, ML algorithms can detect and flag inappropriate content material, making certain a safer and extra user-friendly setting. Actual-time monitoring allows swift motion in opposition to coverage violations, sustaining the integrity and security of the platform. These instruments not solely streamline content material moderation but additionally alleviate the burden on human moderators, enabling them to concentrate on nuanced instances that require human judgment.
- Automated Filtering: ML-powered content material moderation instruments filter and flag inappropriate content material.
- Actual-time Monitoring: Fixed monitoring and swift motion in opposition to coverage violations.
Dynamic Pricing and Income Optimization
Integrating machine studying capabilities into net functions allows dynamic pricing methods and income optimization. By analyzing market traits and consumer conduct, ML algorithms predict optimum pricing methods that maximize income whereas staying aggressive. As an illustration, e-commerce platforms can dynamically modify costs primarily based on demand, growing gross sales potential. These algorithms constantly adapt to altering market circumstances, making certain that pricing methods stay efficient and aligned with enterprise targets.
- Value Prediction: ML predicts optimum pricing methods primarily based on market traits and consumer conduct.
- Income Maximization: Algorithms optimize pricing for elevated gross sales or conversions.
Vitality and Useful resource Effectivity
Machine studying-driven functions contribute to vitality and useful resource effectivity by optimizing consumption. By using algorithms that analyze utilization patterns, these functions optimize useful resource allocation, decreasing vitality consumption and waste. For instance, sensible buildings outfitted with ML-enabled programs can modify vitality utilization primarily based on occupancy patterns, selling sustainability. Furthermore, predictive algorithms anticipate useful resource wants, making certain environment friendly utilization and minimizing pointless consumption.
- Good Useful resource Utilization: ML optimizes vitality and useful resource consumption for eco-friendly operations.
- Useful resource Prediction: Algorithms predict useful resource wants to attenuate wastage and maximize effectivity.
Cross-platform Compatibility and Adaptability
Machine studying capabilities empower net functions to be cross-platform appropriate and adaptive. These functions seamlessly adapt their interfaces and functionalities throughout completely different units and platforms. ML algorithms optimize content material presentation, making certain a constant and user-friendly expertise regardless of the system used. Responsive design parts, pushed by machine studying, make sure that the applying interface adjusts dynamically to various display sizes, providing a easy and intuitive consumer expertise.
- Common Compatibility: ML-driven apps adapt seamlessly throughout varied units and platforms.
- Responsive Design: Algorithms optimize content material presentation for numerous display sizes and interfaces.
Superior-Information Visualization
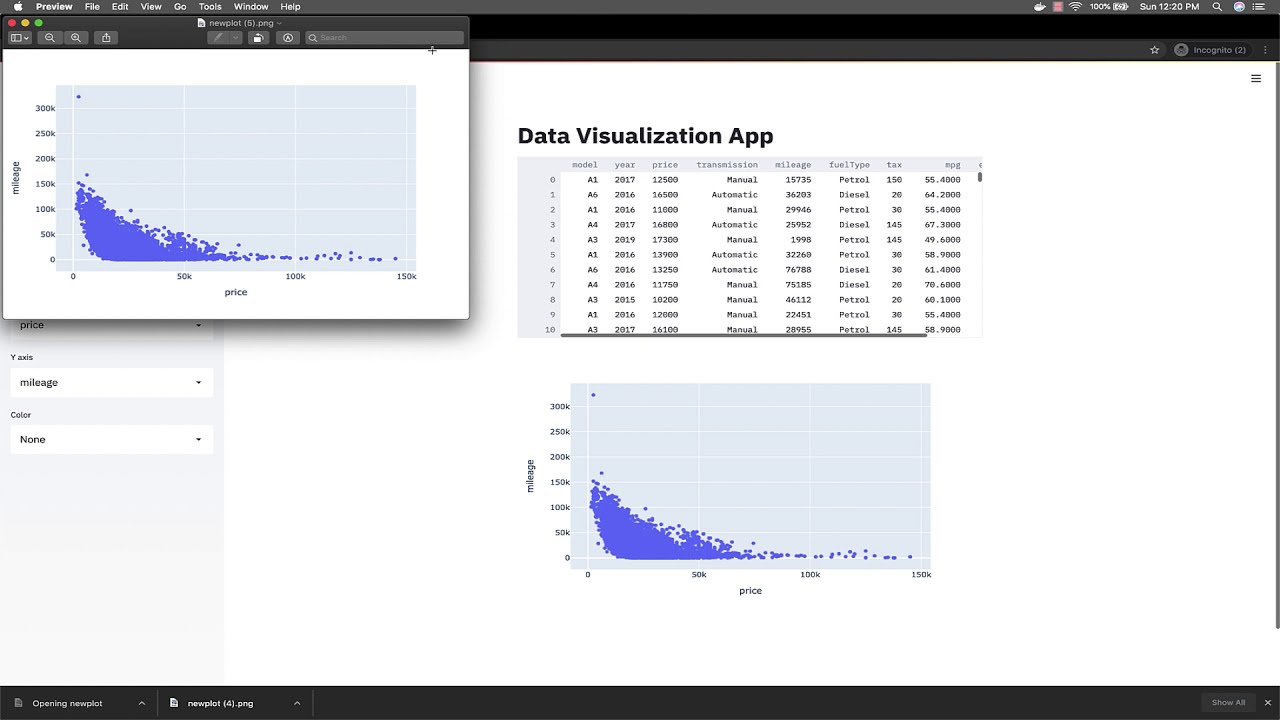
Machine studying integration enhances information visualization inside net functions, providing extra insightful and interactive representations of data. ML-powered instruments can course of complicated datasets and generate visualizations that aren’t solely aesthetically pleasing but additionally present deeper insights. As an illustration, interactive graphs or charts can dynamically modify primarily based on consumer interactions, permitting for a extra personalized exploration of information. These visualizations support customers in comprehending complicated data extra intuitively, fostering higher decision-making and understanding.
- Insightful Illustration: ML-powered information visualization instruments create significant and insightful representations.
- Interactive Visuals: Algorithms generate interactive visualizations for enhanced consumer understanding.
Augmented Consumer Accessibility
Machine studying capabilities facilitate augmented consumer accessibility inside net functions by incorporating options that cater to numerous wants. These functions can make use of ML-driven algorithms to adapt interfaces, making them extra accessible for customers with particular necessities. As an illustration, speech recognition and synthesis applied sciences allow customers with visible impairments to work together with the applying utilizing voice instructions. Adaptive interfaces make sure that customers with completely different accessibility wants can navigate the applying comfortably, selling inclusivity and enhancing general consumer expertise.
- Accessibility Options: ML-driven functions incorporate options for customers with numerous wants.
- Adaptive Interfaces: Algorithms modify interfaces primarily based on consumer preferences or accessibility necessities.
Conclusion
The amalgamation of machine studying capabilities into the framework of next-generation net functions marks a watershed second within the digital panorama. The advantages showcased on this exploration underscore the profound influence that machine studying has on elevating consumer experiences, optimizing operations, and redefining the chances of web-based options.
From hyper-personalized interactions and predictive analytics to enhanced safety measures and adaptive interfaces, the combination of ML not solely augments functionalities but additionally propels industries in direction of innovation and effectivity. The ability of machine studying algorithms empowers net functions to adapt, be taught, and evolve, making a dynamic ecosystem that caters to particular person consumer wants whereas fostering development and sustainability for companies.
As expertise continues to evolve, the synergy between machine studying and net functions holds the promise of continued developments. The continued refinement and integration of ML-driven capabilities will undoubtedly revolutionize how we work together with digital platforms, enabling a extra seamless, clever, and inclusive on-line expertise. Embracing this paradigm shift in direction of clever net functions fortified by machine studying heralds an period the place innovation converges with user-centric design, ushering in a future the place the potential of web-based options is aware of no bounds.
The publish Advantages of Constructing Subsequent-gen Internet Apps With ML Capabilities appeared first on Datafloq.