Editor’s notice: All papers referenced right here symbolize collaborations all through Microsoft and throughout academia and business that embody authors who contribute to Aether, the Microsoft inside advisory physique for AI Ethics and Results in Engineering and Analysis.

-
Video
A human-centered method to AI
Find out how contemplating potential advantages and harms to individuals and society helps create higher AI within the keynote “Challenges and alternatives in accountable AI” (2022 ACM SIGIR Convention on Human Data Interplay and Retrieval).
Synthetic intelligence, like all instruments we construct, is an expression of human creativity. As with all inventive expression, AI manifests the views and values of its creators. A stance that encourages reflexivity amongst AI practitioners is a step towards guaranteeing that AI techniques are human-centered, developed and deployed with the pursuits and well-being of people and society entrance and middle. That is the main focus of analysis scientists and engineers affiliated with Aether, the advisory physique for Microsoft management on AI ethics and results. Central to Aether’s work is the query of who we’re creating AI for—and whether or not we’re creating AI to unravel actual issues with accountable options. With AI capabilities accelerating, our researchers work to know the sociotechnical implications and discover methods to assist on-the-ground practitioners envision and understand these capabilities in keeping with Microsoft AI ideas.
The next is a glimpse into the previous 12 months’s analysis for advancing accountable AI with authors from Aether. All through this work are repeated requires reflexivity in AI practitioners’ processes—that’s, self-reflection to assist us obtain readability about who we’re creating AI techniques for, who advantages, and who might probably be harmed—and for instruments that assist practitioners with the arduous work of uncovering assumptions that will hinder the potential of human-centered AI. The analysis mentioned right here additionally explores crucial parts of accountable AI, resembling being clear about expertise limitations, honoring the values of the individuals utilizing the expertise, enabling human company for optimum human-AI teamwork, bettering efficient interplay with AI, and creating applicable analysis and risk-mitigation methods for multimodal machine studying (ML) fashions.
Contemplating who AI techniques are for
The necessity to domesticate broader views and, for society’s profit, replicate on why and for whom we’re creating AI isn’t solely the accountability of AI growth groups but additionally of the AI analysis group. Within the paper “REAL ML: Recognizing, Exploring, and Articulating Limitations of Machine Studying Analysis,” the authors level out that machine studying publishing usually displays a bias towards emphasizing thrilling progress, which tends to propagate deceptive expectations about AI. They urge reflexivity on the constraints of ML analysis to advertise transparency about findings’ generalizability and potential influence on society—finally, an train in reflecting on who we’re creating AI for. The paper provides a set of guided actions designed to assist articulate analysis limitations, encouraging the machine studying analysis group towards a normal apply of transparency concerning the scope and influence of their work.
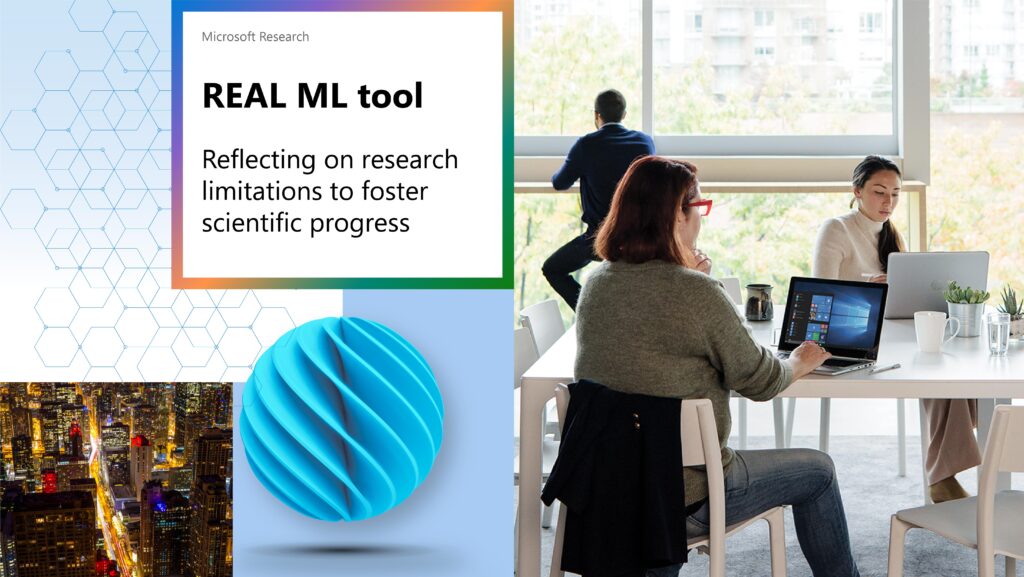
Stroll by means of REAL ML’s tutorial information and worksheet that assist researchers with defining the constraints of their analysis and figuring out societal implications these limitations might have within the sensible use of their work.
Regardless of many organizations formulating ideas to information the accountable growth and deployment of AI, a current survey highlights that there’s a hole between the values prioritized by AI practitioners and people of most of the people. The survey, which included a consultant pattern of the US inhabitants, discovered AI practitioners usually gave much less weight than most of the people to values related to accountable AI. This raises the query of whose values ought to inform AI techniques and shifts consideration towards contemplating the values of the individuals we’re designing for, aiming for AI techniques which are higher aligned with individuals’s wants.
Associated papers
Creating AI that empowers human company
Supporting human company and emphasizing transparency in AI techniques are confirmed approaches to constructing applicable belief with the individuals techniques are designed to assist. In human-AI teamwork, interactive visualization instruments can allow individuals to capitalize on their very own area experience and allow them to simply edit state-of-the-art fashions. For instance, physicians utilizing GAM Changer can edit danger prediction fashions for pneumonia and sepsis to include their very own medical information and make higher remedy choices for sufferers.
A examine analyzing how AI can enhance the worth of quickly rising citizen-science contributions discovered that emphasizing human company and transparency elevated productiveness in an internet workflow the place volunteers present useful info to assist AI classify galaxies. When selecting to decide in to utilizing the brand new workflow and receiving messages that pressured human help was obligatory for troublesome classification duties, members have been extra productive with out sacrificing the standard of their enter they usually returned to volunteer extra usually.
Failures are inevitable in AI as a result of no mannequin that interacts with the ever-changing bodily world could be full. Human enter and suggestions are important to decreasing dangers. Investigating reliability and security mitigations for techniques resembling robotic field pushing and autonomous driving, researchers formalize the issue of unfavorable unintended effects (NSEs), the undesirable habits of those techniques. The researchers experimented with a framework during which the AI system makes use of quick human help within the type of suggestions—both concerning the person’s tolerance for an NSE prevalence or their determination to switch the surroundings. Outcomes reveal that AI techniques can adapt to efficiently mitigate NSEs from suggestions, however amongst future concerns, there stays the problem of creating methods for gathering correct suggestions from people utilizing the system.
The purpose of optimizing human-AI complementarity highlights the significance of partaking human company. In a large-scale examine analyzing how bias in fashions influences people’ choices in a job recruiting process, researchers made a stunning discovery: when working with a black-box deep neural community (DNN) recommender system, individuals made considerably fewer gender-biased choices than when working with a bag-of-words (BOW) mannequin, which is perceived as extra interpretable. This implies that individuals are likely to replicate and depend on their very own judgment earlier than accepting a suggestion from a system for which they’ll’t comfortably kind a psychological mannequin of how its outputs are derived. Researchers name for exploring methods to raised interact human reflexivity when working with superior algorithms, which could be a means for bettering hybrid human-AI decision-making and mitigating bias.
How we design human-AI interplay is essential to complementarity and empowering human company. We have to rigorously plan how individuals will work together with AI techniques which are stochastic in nature and current inherently totally different challenges than deterministic techniques. Designing and testing human interplay with AI techniques as early as attainable within the growth course of, even earlier than groups spend money on engineering, might help keep away from pricey failures and redesign. Towards this purpose, researchers suggest early testing of human-AI interplay by means of factorial surveys, a technique from the social sciences that makes use of quick narratives for deriving insights about individuals’s perceptions.
However testing for optimum person expertise earlier than groups spend money on engineering could be difficult for AI-based options that change over time. The continued nature of an individual adapting to a always updating AI function makes it troublesome to look at person habits patterns that may inform design enhancements earlier than deploying a system. Nevertheless, experiments reveal the potential of HINT (Human-AI INtegration Testing), a framework for uncovering over-time patterns in person habits throughout pre-deployment testing. Utilizing HINT, practitioners can design take a look at setup, acquire knowledge through a crowdsourced workflow, and generate studies of user-centered and offline metrics.
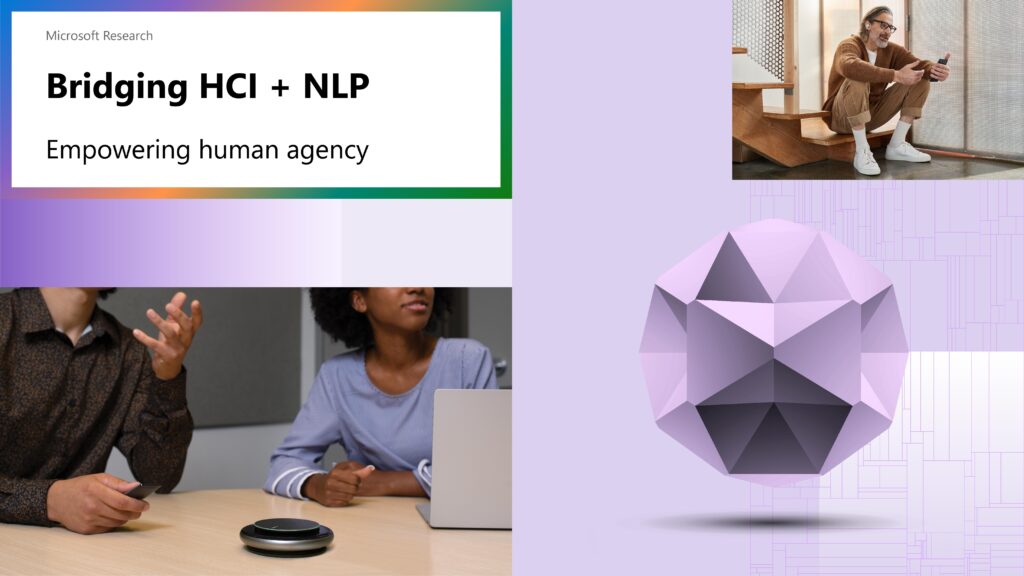
Take a look at the 2022 anthology of this annual workshop that brings human-computer interplay (HCI) and pure language processing (NLP) analysis collectively for bettering how individuals can profit from NLP apps they use each day.
Associated papers
Though we’re nonetheless within the early phases of understanding responsibly harness the potential of huge language and multimodal fashions that can be utilized as foundations for constructing quite a lot of AI-based techniques, researchers are creating promising instruments and analysis methods to assist on-the-ground practitioners ship accountable AI. The reflexivity and assets required for deploying these new capabilities with a human-centered method are essentially suitable with enterprise objectives of strong providers and merchandise.
Pure language era with open-ended vocabulary has sparked plenty of creativeness in product groups. Challenges persist, nonetheless, together with for bettering poisonous language detection; content material moderation instruments usually over-flag content material that mentions minority teams with out respect to context whereas lacking implicit toxicity. To assist deal with this, a new large-scale machine-generated dataset, ToxiGen, permits practitioners to fine-tune pretrained hate classifiers for bettering detection of implicit toxicity for 13 minority teams in each human- and machine-generated textual content.
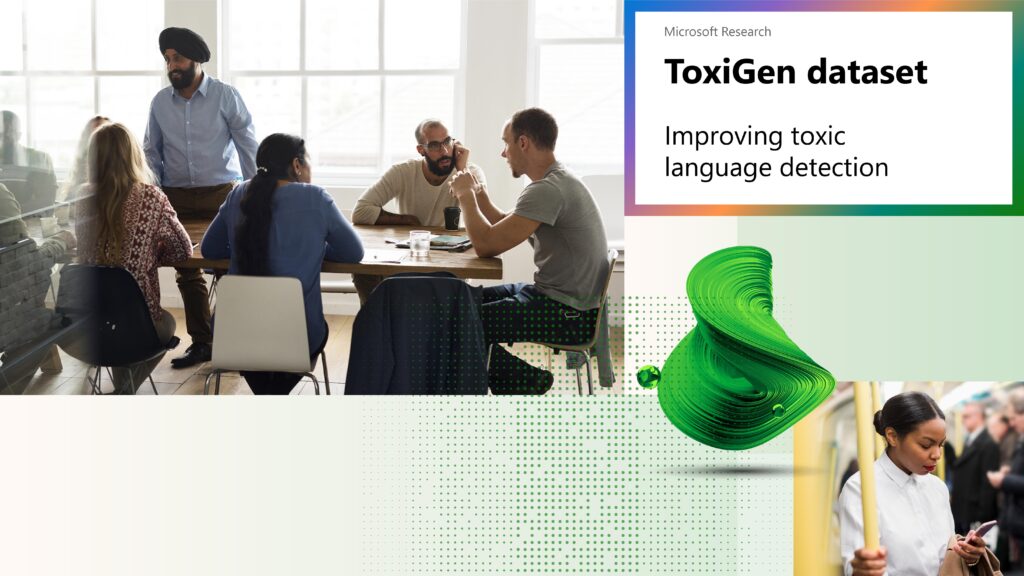
Obtain the large-scale machine-generated ToxiGen dataset and set up supply code for fine-tuning poisonous language detection techniques for adversarial and implicit hate speech for 13 demographic minority teams. Supposed for analysis functions.
Multimodal fashions are proliferating, resembling people who mix pure language era with pc imaginative and prescient for providers like picture captioning. These advanced techniques can floor dangerous societal biases of their output and are difficult to judge for mitigating harms. Utilizing a state-of-the-art picture captioning service with two widespread image-captioning datasets, researchers isolate the place within the system fairness-related harms originate and current a number of measurement methods for 5 particular kinds of representational hurt: denying individuals the chance to self-identify, reifying social teams, stereotyping, erasing, and demeaning.
The business creation of AI-powered code mills has launched novice builders alongside professionals to massive language mannequin (LLM)-assisted programming. An outline of the LLM-assisted programming expertise reveals distinctive concerns. Programming with LLMs invitations comparability to associated methods of programming, resembling search, compilation, and pair programming. Whereas there are certainly similarities, the empirical studies counsel it’s a distinct means of programming with its personal distinctive mix of behaviors. For instance, extra effort is required to craft prompts that generate the specified code, and programmers should examine the instructed code for correctness, reliability, security, and safety. Nonetheless, a person examine analyzing what programmers worth in AI code era reveals that programmers do discover worth in instructed code as a result of it’s simple to edit, rising productiveness. Researchers suggest a hybrid metric that mixes useful correctness and similarity-based metrics to finest seize what programmers worth in LLM-assisted programming, as a result of human judgment ought to decide how a expertise can finest serve us.
Associated papers
Understanding and supporting AI practitioners
Organizational tradition and enterprise objectives can usually be at odds with what practitioners want for mitigating equity and different accountable AI points when their techniques are deployed at scale. Accountable, human-centered AI requires a considerate method: simply because a expertise is technically possible doesn’t imply it ought to be created.
Equally, simply because a dataset is on the market doesn’t imply it’s applicable to make use of. Understanding why and the way a dataset was created is essential for serving to AI practitioners determine on whether or not it ought to be used for his or her functions and what its implications are for equity, reliability, security, and privateness. A examine specializing in how AI practitioners method datasets and documentation reveals present practices are casual and inconsistent. It factors to the want for knowledge documentation frameworks designed to suit inside practitioners’ present workflows and that clarify the accountable AI implications of utilizing a dataset. Primarily based on these findings, researchers iterated on Datasheets for Datasets and proposed the revised Aether Information Documentation Template.
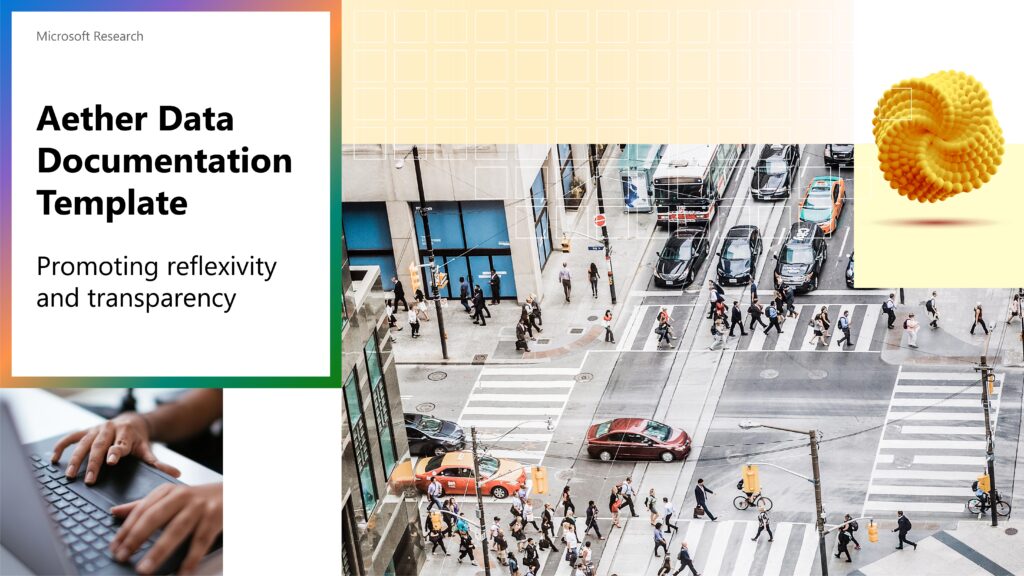
Use this versatile template to replicate and assist doc underlying assumptions, potential dangers, and implications of utilizing your dataset.
AI practitioners discover themselves balancing the pressures of delivering to fulfill enterprise objectives and the time necessities obligatory for the accountable growth and analysis of AI techniques. Analyzing these tensions throughout three expertise corporations, researchers carried out interviews and workshops to study what practitioners want for measuring and mitigating AI equity points amid time strain to launch AI-infused merchandise to wider geographic markets and for extra various teams of individuals. Contributors disclosed challenges in gathering applicable datasets and discovering the suitable metrics for evaluating how pretty their system will carry out after they can’t establish direct stakeholders and demographic teams who will likely be affected by the AI system in quickly broadening markets. For instance, hate speech detection will not be enough throughout cultures or languages. A take a look at what goes into AI practitioners’ choices round what, when, and consider AI techniques that use pure language era (NLG) additional emphasizes that when practitioners don’t have readability about deployment settings, they’re restricted in projecting failures that might trigger particular person or societal hurt. Past issues for detecting poisonous speech, different problems with equity and inclusiveness—for instance, erasure of minority teams’ distinctive linguistic expression—are hardly ever a consideration in practitioners’ evaluations.
Dealing with time constraints and competing enterprise goals is a actuality for groups deploying AI techniques. There are various alternatives for creating built-in instruments that may immediate AI practitioners to assume by means of potential dangers and mitigations for sociotechnical techniques.
Associated papers
Serious about it: Reflexivity as an important for society and business objectives
As we proceed to examine what all is feasible with AI’s potential, one factor is evident: creating AI designed with the wants of individuals in thoughts requires reflexivity. Now we have been excited about human-centered AI as being targeted on customers and stakeholders. Understanding who we’re designing for, empowering human company, bettering human-AI interplay, and creating hurt mitigation instruments and methods are as essential as ever. However we additionally want to show a mirror towards ourselves as AI creators. What values and assumptions will we carry to the desk? Whose values get to be included and whose are ignored? How do these values and assumptions affect what we construct, how we construct, and for whom? How can we navigate advanced and demanding organizational pressures as we endeavor to create accountable AI? With applied sciences as highly effective as AI, we will’t afford to be targeted solely on progress for its personal sake. Whereas we work to evolve AI applied sciences at a quick tempo, we have to pause and replicate on what it’s that we’re advancing—and for whom.