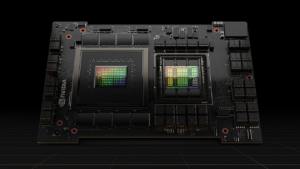
Firms that toyed round with generative AI this 12 months can be trying to play for retains in 2024 with manufacturing GenAI apps that transfer the enterprise needle. Contemplating there’s not sufficient GPUs to go round now, the place will firms discover sufficient compute to satisfy hovering demand? Because the world’s largest information middle operator, AWS is working to satisfy compute demand in a number of methods, in line with Chetan Kapoor, the corporate’s director of product administration for EC2.
Most of AWS’s plans to satisfy future GenAI compute calls for contain its companion, GPU-maker Nvidia. When the launch of ChatGPT sparked the GenAI revolution a 12 months in the past, it spurred a run on high-end A100 Hopper chips which can be wanted to coach massive language fashions (LLMs). Whereas a scarcity of A100s has made it tough for smaller companies to acquire the GPUs, AWS has the good thing about having a particular relationship with the chipmaker, which has enabled it to carry “ultra-clusters” on-line.
“Our partnership with Nvidia has been very, very robust,” Kapoor informed Datanami at AWS’s current re:Invent convention in Las Vegas, Nevada. “This Nvidia expertise is simply phenomenal. We predict it’s going to be a giant enabler for the upcoming foundational fashions which can be going to energy GenAI going ahead. We’re collaborating with Nvidia not solely on the rack degree, however on the cluster degree.”
About three years in the past, AWS launched its p4 situations, which allows customers to lease entry to GPUs. Since basis fashions are so massive, it sometimes requires a lot of GPUs to be linked collectively for coaching. That’s the place the idea of GPU ultra-clusters got here from.
“We have been seeing clients go from consuming tens of GPUs to lots of to hundreds, they usually wanted for all of those GPUs to be co-located in order that they may seamlessly use all of them in a single mixed coaching job,” Kapoor stated.
At re:Invent, AWS CEO Adam Selipskyi and Nvidia CEO Jensen Huang introduced that AWS can be constructing an enormous new ultra-cluster composed of 16,000 Nvidia H200 GPUs and a few variety of AWS Trainium2 chips lashed collectively utilizing AWS’s Ethernet-based Elastic Material Adapter (EFA) networking expertise. The ultra-cluster, which can sport 65 exaflops of compute capability, is slated to go surfing in certainly one of AWS’s information facilities in 2024.
Nvidia goes to be one of many foremost tenants on this new ultra-cluster for its AI modeling service, and AWS may also have entry to this capability for its numerous GenAI providers, together with Titan and Bedrock LLM choices. In some unspecified time in the future, common AWS customers may also get entry to that ultra-cluster, or presumably further ultra-clusters, for their very own AI use instances, Kapoor stated.
“That that 16,000 deployment is meant to be a single cluster, however past that, it would most likely break up out into different chunks of clusters,” he stated. “There is likely to be one other 8,000 that you just may deploy, or a bit of 4,000 relying on the particular geography and what clients are on the lookout for.”
Kapoor, who’s a part of the senior workforce overseeing EC2 at AWS, stated he couldn’t touch upon the precise GPU capability that will launched to common AWS clients, however he assured this reporter that it might be substantial.
“Sometimes, once we purchase from Nvidia, it’s not within the hundreds,” he stated, indicating it was larger. “It’s a fairly vital consumption from our aspect, whenever you have a look at what we’ve got executed on A100s and what we’re doing actively on H100s, so it’s going to be a big deployment.”
Nevertheless, contemplating the insatiable demand for GPUs, until in some way curiosity in GenAI falls off a cliff, it’s clear that AWS and each different cloud supplier can be oversubscribed on the subject of GPU demand versus GPU capability. That’s one of many the reason why AWS is on the lookout for different suppliers of AI compute capability in addition to Nvidia.
“We’ll proceed to be within the face of experimenting, attempting out various things,” Kapoor stated. “However we all the time have our eyes open. If there’s new expertise that someone else innovating, if there’s a brand new chip sort that’s tremendous, tremendous relevant in a selected vertical, we’re very happy to have a look and determine a strategy to make them out there within the AWS ecosystem.”
It is also constructing its personal silicon. As an illustration, there may be the aforementioned Trainium chip. At re:invent, AWS introduced the successor chip, Trainium2, will ship a 4x efficiency increase over the primary gen Trainium chip, making it helpful for coaching the biggest basis fashions, with lots of of billions and even trillions of parameters, the corporate stated.
Trainium1 has been usually out there for over a 12 months, and is being utilized in many ultra-clusters, Kapoor stated, the largest of which has 30,000 nodes. Trainium2, in the meantime, can be deployed in ultra-clusters with as much as 100,000 nodes, which can be used to coach the subsequent era of huge language fashions.
“We’re capable of help coaching of 100 to 150 billion parameter measurement fashions very, very successfully [with Trainium1] and we’ve got software program help for coaching massive fashions coming shortly within the subsequent few months,” Kapoor stated. “There’s nothing basic within the {hardware} design that limits how massive of a mannequin [it can train]. It’s principally a scale-out downside. It’s not how massive a selected chip is–it’s what number of of them you’ll be able to really get operational in parallel and do a extremely efficient job in spreading your coaching workload throughout them.”
Nevertheless, Trainium2 received’t be able to do helpful work for months. Within the meantime, AWS is trying to different technique of liberating up compute sources for giant mannequin coaching. That features the brand new GPU capability block EC2 situations AWS unveiled at re:Invent.
With the capability block mannequin, clients primarily make reservations for GPU compute weeks or months forward of time. The hope is that it’s going to persuade AWS clients to cease hoarding unused GPU capability for worry they received’t get it again, Kapoor stated.
“It’s frequent data [that] there aren’t sufficient GPUs within the business to go round,” he stated. “So we’re seeing patterns the place there are some clients which can be stepping into, consuming capability from us, and simply holding on to it for an prolonged time frame due to the worry that in the event that they launch that capability, they won’t get it again once they want it.”
Massive firms can afford to waste 30% of their GPU capability so long as they’re getting good AI mannequin coaching returns from the opposite 70%, he stated. However that compute mannequin doesn’t fly for smaller firms.
“This was an answer that’s geared to sort of clear up that downside the place small to medium enterprises have a predictable means of buying capability they usually can really plan round it,” he stated. “So if a workforce is engaged on a brand new machine studying mannequin they usually’re like, OK, we’re going to be prepared in two weeks to truly go all out and do a big coaching run, I need to go and make it possible for I’ve the capability for it to have that confidence that that capability can be out there.”
AWS isn’t wedded to Nvidia, and has bought AI coaching chips from Intel too. Buyer can get entry to Intel Gaudi accelerators through the EC2 DL1 situations, which provide eight Gaudi accelerators with 32 GB of excessive bandwidth reminiscence (HBM) per accelerator, 768 GB of system reminiscence, second-gen Intel Xeon Scalable processors, 400 Gbps of networking throughput, and 4 TB of native NVMe storage, in line with AWS’s DL1 webpage.
Kapoor additionally stated AWS is open to GPU innovation from AMD. Final week, that chipmaker launched the much-anticipated MI300X GPU, which provides some efficiency benefits over the H100 and upcoming H200 GPUs from Nvidia.
“It’s positively on the radar,” Kapoor stated of AMD normally in late November, greater than every week earlier than the MI300X launch. “The factor to be aware of is it’s not simply the silicon efficiency. It’s additionally software program compatibility and the way straightforward to make use of it’s for builders.”
On this GenAI mad sprint, some clients are delicate to how rapidly they’ll iterate and are greater than prepared to spend extra money if it means they’ll get to market faster, Kapoor stated.
“But when there are different options that really give them that capability to innovate rapidly whereas saving 30%, 40%, they may definitely take that,” he stated. “I feel there’s an honest quantity of labor for everyone else outdoors of Nvidia to sharpen up their software program capabilities and ensure they’re making it tremendous, tremendous straightforward for purchasers emigrate from what they’re doing at the moment to a selected platform and vice versa.”
However coaching AI fashions is barely half the battle. Operating the AI mannequin in manufacturing additionally requires massive quantities of compute, often of the GPU selection. There are some indications that the quantity of compute required by inference workloads really exceeds the compute demand on the preliminary coaching run. As GenAI workloads come on-line, that makes the present GPU squeeze even worse.
“Even for inference, you really need accelerated compute, like GPUs or Inferential or Trainiums within the again,” Kapoor stated. “In the event you’re interacting with a chatbot and also you ship it a message and it’s a must to wait seconds for it correspond again, it’s only a horrible consumer expertise. Irrespective of how correct or good of a response that it’s, if it takes too lengthy for it to reply again, you’re going to lose your buyer.”
Kapoor famous that Nvidia is engaged on a brand new GPU structure that may supply higher inference capability. The introduction of a brand new 8-bit floating level information sort, FP8, may also ease the capability crunch. AWS can be taking a look at FP8, he stated.
“Once we are innovating on constructing customized chips, we’re very, very keenly conscious of the coaching in addition to inference necessities, so if someone is definitely deploying these fashions in manufacturing, we need to be certain that we run them as successfully from an influence and a compute standpoint,” Kapoor stated. “We’ve just a few clients which can be really in manufacturing [with GenAI], they usually have been in manufacturing for a number of months now. However the overwhelming majority of enterprises are nonetheless within the technique of sort of determining tips on how to reap the benefits of these GenAI capabilities.”
As firms get nearer to going into manufacturing with GenAI, they may think about these inferencing prices and search for methods to optimize the fashions, Kapoor stated. As an illustration, there are distillation strategies that may shrink the compute calls for for inference workloads relative to coaching, he stated. Quantization is one other methodology that can be known as upon to make GenAI make financial sense. However we’re not there but.
“What we’re seeing proper now’s simply a whole lot of eagerness for folks to get an answer out out there,” he stated. “Of us haven’t gotten to some extent, or should not prioritizing value manufacturing and financial optimization at this explicit time limit. They’re simply being like, okay, what is that this expertise able to doing? How can I take advantage of it to reinvent or present a greater expertise for my builders or clients? After which sure, they’re aware that sooner or later, if this actually takes off and it’s actually impactful kind a enterprise standpoint, they’ll have to come back again in and begin to optimize value.”
Associated Objects:
Inside AWS’s Plans to Make S3 Quicker and Higher
AWS Provides Vector Capabilities to Extra Databases
AWS Teases 65 Exaflop ‘Extremely-Cluster’ with Nvidia, Launches New Chips