You’re on the wheel of your automobile however you’re exhausted. Your shoulders begin to sag, your neck begins to droop, your eyelids slide down. As your head pitches ahead, you swerve off the highway and velocity by way of a subject, crashing right into a tree.
However what in case your automobile’s monitoring system recognised the tell-tale indicators of drowsiness and prompted you to drag off the highway and park as a substitute? The European Fee has legislated that from this 12 months, new autos be fitted with methods to catch distracted and sleepy drivers to assist avert accidents. Now numerous startups are coaching synthetic intelligence methods to recognise the giveaways in our facial expressions and physique language.
These firms are taking a novel strategy for the sphere of AI. As an alternative of filming hundreds of real-life drivers falling asleep and feeding that info right into a deep-learning mannequin to “be taught” the indicators of drowsiness, they’re creating thousands and thousands of faux human avatars to re-enact the sleepy indicators.
“Massive knowledge” defines the sphere of AI for a cause. To coach deep studying algorithms precisely, the fashions have to have a mess of knowledge factors. That creates issues for a process corresponding to recognising an individual falling asleep on the wheel, which might be tough and time-consuming to movie occurring in hundreds of automobiles. As an alternative, firms have begun constructing digital datasets.
Synthesis AI and Datagen are two firms utilizing full-body 3D scans, together with detailed face scans, and movement knowledge captured by sensors positioned everywhere in the physique, to collect uncooked knowledge from actual folks. This knowledge is fed by way of algorithms that tweak varied dimensions many instances over to create thousands and thousands of 3D representations of people, resembling characters in a online game, participating in numerous behaviours throughout quite a lot of simulations.
Within the case of somebody falling asleep on the wheel, they may movie a human performer falling asleep and mix it with movement seize, 3D animations and different strategies used to create video video games and animated motion pictures, to construct the specified simulation. “You possibly can map [the target behaviour] throughout hundreds of various physique sorts, totally different angles, totally different lighting, and add variability into the motion as nicely,” says Yashar Behzadi, CEO of Synthesis AI.
Utilizing artificial knowledge cuts out lots of the messiness of the extra conventional strategy to practice deep studying algorithms. Usually, firms must amass an enormous assortment of real-life footage and low-paid staff would painstakingly label every of the clips. These could be fed into the mannequin, which might learn to recognise the behaviours.
The large promote for the artificial knowledge strategy is that it’s faster and cheaper by a large margin. However these firms additionally declare it could possibly assist sort out the bias that creates an enormous headache for AI builders. It’s nicely documented that some AI facial recognition software program is poor at recognising and accurately figuring out specific demographic teams. This tends to be as a result of these teams are underrepresented within the coaching knowledge, that means the software program is extra prone to misidentify these folks.
Niharika Jain, a software program engineer and knowledgeable in gender and racial bias in generative machine studying, highlights the infamous instance of Nikon Coolpix’s “blink detection” characteristic, which, as a result of the coaching knowledge included a majority of white faces, disproportionately judged Asian faces to be blinking. “ driver-monitoring system should keep away from misidentifying members of a sure demographic as asleep extra usually than others,” she says.
The everyday response to this drawback is to collect extra knowledge from the underrepresented teams in real-life settings. However firms corresponding to Datagen say that is not essential. The corporate can merely create extra faces from the underrepresented teams, that means they’ll make up a much bigger proportion of the ultimate dataset. Actual 3D face scan knowledge from hundreds of individuals is whipped up into thousands and thousands of AI composites. “There’s no bias baked into the information; you have got full management of the age, gender and ethnicity of the folks that you just’re producing,” says Gil Elbaz, co-founder of Datagen. The creepy faces that emerge don’t seem like actual folks, however the firm claims that they’re comparable sufficient to show AI methods how to reply to actual folks in comparable eventualities.
There’s, nonetheless, some debate over whether or not artificial knowledge can actually remove bias. Bernease Herman, an information scientist on the College of Washington eScience Institute, says that though artificial knowledge can enhance the robustness of facial recognition fashions on underrepresented teams, she doesn’t consider that artificial knowledge alone can shut the hole between the efficiency on these teams and others. Though the businesses typically publish tutorial papers showcasing how their algorithms work, the algorithms themselves are proprietary, so researchers can not independently consider them.
In areas corresponding to digital actuality, in addition to robotics, the place 3D mapping is vital, artificial knowledge firms argue it might truly be preferable to coach AI on simulations, particularly as 3D modelling, visible results and gaming applied sciences enhance. “It’s solely a matter of time till… you may create these digital worlds and practice your methods utterly in a simulation,” says Behzadi.
This sort of considering is gaining floor within the autonomous automobile business, the place artificial knowledge is turning into instrumental in educating self-driving autos’ AI tips on how to navigate the highway. The standard strategy – filming hours of driving footage and feeding this right into a deep studying mannequin – was sufficient to get automobiles comparatively good at navigating roads. However the subject vexing the business is tips on how to get automobiles to reliably deal with what are often called “edge instances” – occasions which are uncommon sufficient that they don’t seem a lot in thousands and thousands of hours of coaching knowledge. For instance, a baby or canine working into the highway, difficult roadworks and even some visitors cones positioned in an surprising place, which was sufficient to stump a driverless Waymo automobile in Arizona in 2021.
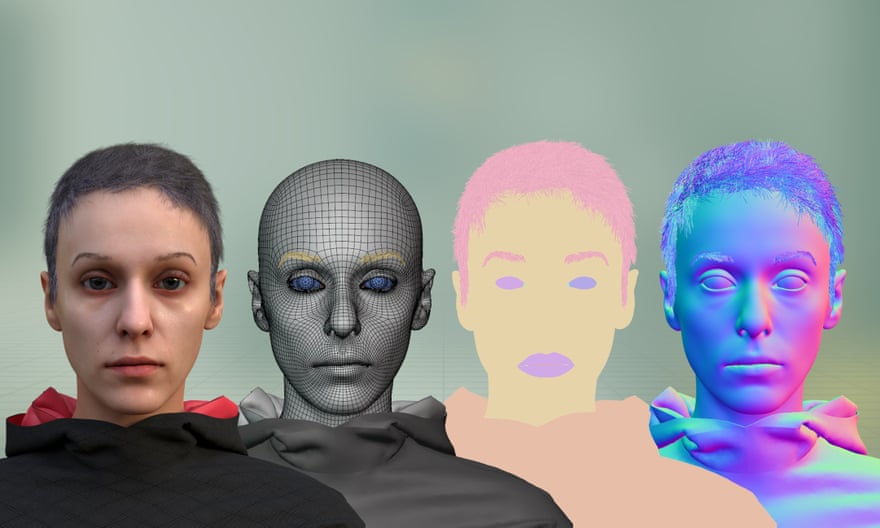
With artificial knowledge, firms can create infinite variations of eventualities in digital worlds that not often occur in the true world. “As an alternative of ready thousands and thousands extra miles to build up extra examples, they’ll artificially generate as many examples as they want of the sting case for coaching and testing,” says Phil Koopman, affiliate professor in electrical and pc engineering at Carnegie Mellon College.
AV firms corresponding to Waymo, Cruise and Wayve are more and more counting on real-life knowledge mixed with simulated driving in digital worlds. Waymo has created a simulated world utilizing AI and sensor knowledge collected from its self-driving autos, full with synthetic raindrops and photo voltaic glare. It makes use of this to coach autos on regular driving conditions, in addition to the trickier edge instances. In 2021, Waymo advised the Verge that it had simulated 15bn miles of driving, versus a mere 20m miles of actual driving.
An additional advantage to testing autonomous autos out in digital worlds first is minimising the possibility of very actual accidents. “A big cause self-driving is on the forefront of lots of the artificial knowledge stuff is fault tolerance,” says Herman. “A self-driving automobile making a mistake 1% of the time, and even 0.01% of the time, might be an excessive amount of.”
In 2017, Volvo’s self-driving expertise, which had been taught how to reply to massive North American animals corresponding to deer, was baffled when encountering kangaroos for the primary time in Australia. “If a simulator doesn’t learn about kangaroos, no quantity of simulation will create one till it’s seen in testing and designers determine tips on how to add it,” says Koopman. For Aaron Roth, professor of pc and cognitive science on the College of Pennsylvania, the problem can be to create artificial knowledge that’s indistinguishable from actual knowledge. He thinks it’s believable that we’re at that time for face knowledge, as computer systems can now generate photorealistic photographs of faces. “However for lots of different issues,” – which can or might not embody kangaroos – “I don’t assume that we’re there but.”