As an information scientist, among the finest issues about working with DataRobot prospects is the sheer number of extremely fascinating questions that come up. Just lately, a potential buyer requested me how I reconcile the truth that DataRobot has a number of very profitable funding banks utilizing DataRobot to reinforce the P&L of their buying and selling companies with my feedback that machine studying fashions aren’t all the time nice at predicting monetary asset costs. Peek into our dialog to study when machine studying does—and doesn’t—work properly in monetary markets use circumstances.
Why is machine studying in a position to carry out properly in excessive frequency buying and selling purposes, however is so dangerous at predicting asset costs longer-term?
Whereas there have been some successes within the business utilizing machine studying for value prediction, they’ve been few and much between. As a rule of thumb, the shorter the prediction time horizon, the higher the chances of success.
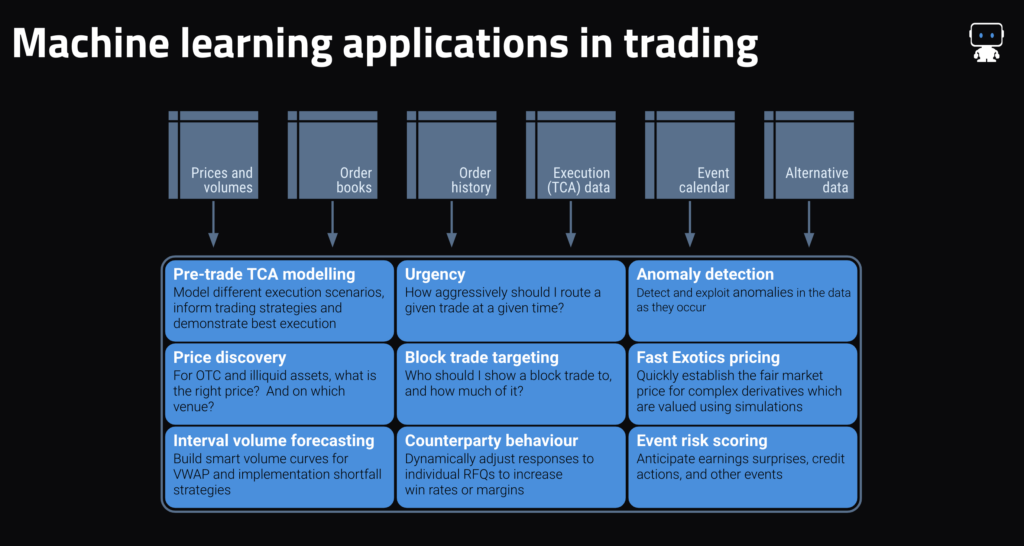
Usually talking, market making use circumstances that DataRobot (and different machine studying approaches) excel at share a number of of the next traits:
- For ahead value prediction: a really brief prediction horizon (usually throughout the subsequent one to 10 seconds), the supply of excellent order ebook knowledge, and an acknowledgment that even a mannequin that’s 55%–60% correct is helpful—it’s in the end a share recreation.
- For value discovery (e.g., establishing an acceptable value illiquid securities, predicting the place liquidity might be situated, and figuring out acceptable hedge ratios) in addition to extra typically: the existence of excellent historic commerce knowledge on the belongings to be priced (e.g., TRACE, Asian bond market reporting, ECNs’ commerce historical past) in addition to a transparent set of extra liquid belongings which can be utilized as predictors (e.g., extra liquid credit, bond futures, swaps markets, and so forth.).
- For counterparty habits prediction: some type of structured knowledge which comprises not solely gained trades but additionally unsuccessful requests/responses.
- Throughout purposes: an data edge, as an example from commanding a big share of the stream in that asset class, or from having buyer habits knowledge that can be utilized.
Areas the place any type of machine studying will battle are usually characterised by a number of of those points:
- Quickly altering regimes, behaviors and drivers: a key cause why longer-term predictions are so laborious. We fairly often discover that the important thing mannequin drivers change very frequently in most monetary markets, with a variable that’s a helpful indicator for one week or month having little data content material within the subsequent. Even in profitable purposes, fashions are re-trained and re-deployed very frequently (usually at the very least weekly).
- Rare knowledge: a basic instance right here is month-to-month or much less frequent knowledge. In such circumstances, the habits being modeled usually adjustments so usually that by the point that sufficient coaching knowledge for machine studying has accrued (24 months or above), the market is in a special regime. For what it’s price, a couple of of our prospects have certainly had some success at, as an example, inventory choice utilizing predictions on a one-month horizon, however they’re (understandably) not telling us how they’re doing it.
- Sparse knowledge: the place there’s inadequate knowledge out there to get a superb image of the market in mixture, akin to sure OTC markets the place there aren’t any good ECNs.
- An absence of predictors: on the whole, knowledge on previous habits of the variable being predicted (e.g., costs) isn’t sufficient. You additionally want knowledge describing the drivers of that variable (e.g., order books, flows, expectations, positioning). Previous efficiency isn’t indicative of future outcomes… .
- Restricted historical past of comparable regimes: as a result of machine studying fashions are all about recognising patterns in historic knowledge, new markets or belongings could be very troublesome for ML fashions. That is recognized in academia because the “chilly begin downside.” There are numerous methods to take care of it, however none of them are good.
- Not truly being a machine studying downside: Worth-at-Danger modeling is the basic instance right here—VaR isn’t a prediction of something, it’s a statistical summation of simulation outcomes. That mentioned, predicting the result of a simulation is an ML downside, and there are some good ML purposes in pricing complicated, path-dependent derivatives.
Lastly, and apart from the above, a essential success think about any machine studying use case which shouldn’t be underestimated is the involvement of succesful and motivated individuals (usually quants and typically knowledge scientists) who perceive the info (and the way to manipulate it), enterprise processes, and worth levers. Success is normally pushed by such individuals finishing up many iterative experiments on the issue at hand, which is in the end the place our platform is available in. As mentioned, we massively speed up that means of experimentation. There’s lots that may be automated in machine studying, however area information can’t be.
To summarize: it’s truthful to say that the chance of success in buying and selling use circumstances is positively correlated with the frequency of the buying and selling (or at the very least negatively with the holding interval/horizon) with a couple of exceptions to show the rule. It’s additionally price allowing for that machine studying is usually higher at second-order use circumstances akin to predicting the drivers of markets, as an example, occasion threat and, to some extent, volumes, fairly than first-order value predictions— topic to the above caveats.
In regards to the writer

Managing Director, Monetary Markets Information Science
Peter leads DataRobot’s monetary markets knowledge science follow and works intently with fintech, banking, and asset administration purchasers on quite a few high-ROI use circumstances for the DataRobot AI Platform. Previous to becoming a member of DataRobot, he gained twenty-five years’ expertise in senior quantitative analysis, portfolio administration, buying and selling, threat administration and knowledge science roles at funding banks and asset managers together with Morgan Stanley, Warburg Pincus, Goldman Sachs, Credit score Suisse, Lansdowne Companions and Invesco, in addition to spending a number of years as a accomplice at a start-up international equities hedge fund. Peter has an M.Sc. in Information Science from Metropolis, College of London, an MBA from Cranfield College College of Administration, and a B.Sc. in Accounting and Monetary Evaluation from the College of Warwick. His paper, “Looking Excessive and Low: Visualising Shifting Correlations in Monetary Markets”, was revealed within the July 2018 situation of Pc Graphics Discussion board.