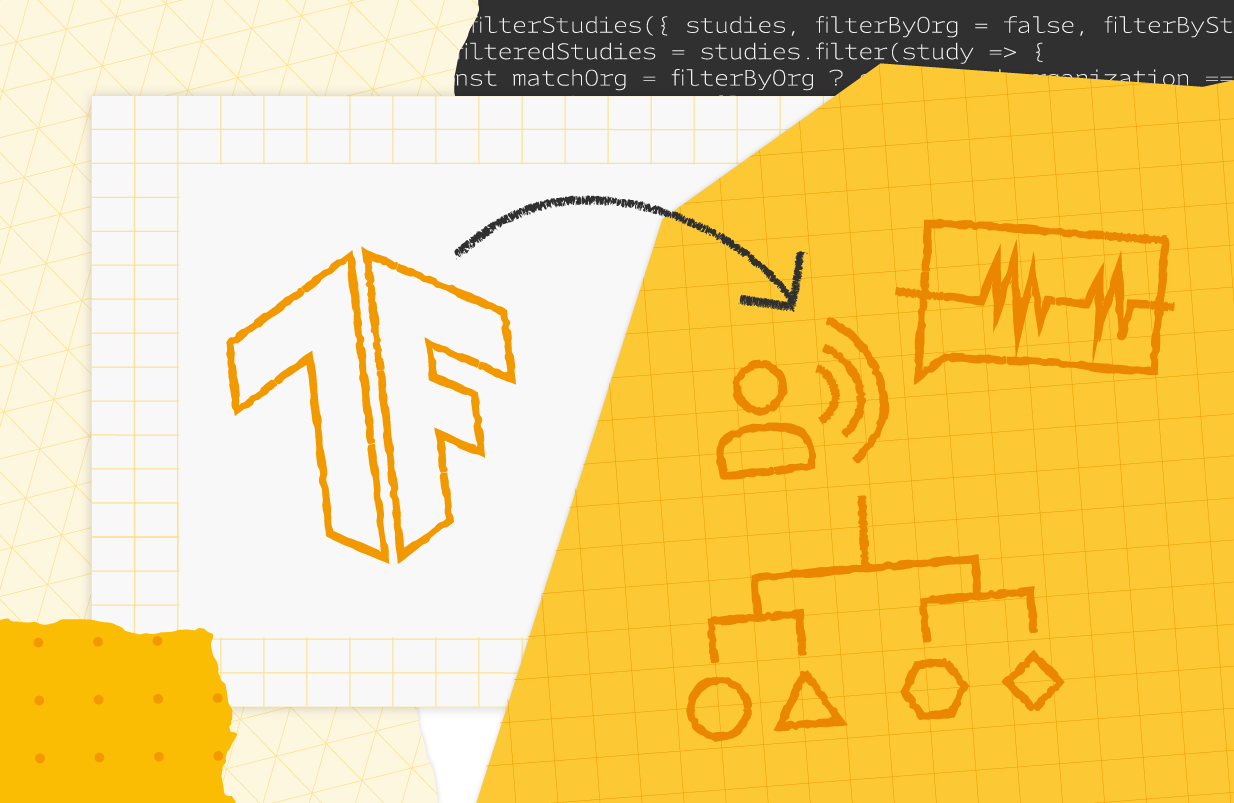
Posted by Paul McCartney, Software program Engineer, Vivek Kwatra, Analysis Scientist, Yu Zhang, Analysis Scientist, Brian Colonna, Software program Engineer, and Mor Miller, Software program Engineer
Folks more and more look to video as their most well-liked approach to be higher knowledgeable, to discover their pursuits, and to be entertained. And but a video’s spoken language is usually a barrier to understanding. For instance, a excessive share of YouTube movies are in English however lower than 20% of the world’s inhabitants speaks English as their first or second language. Voice dubbing is more and more getting used to remodel video into different languages, by translating and changing a video’s authentic spoken dialogue. That is efficient in eliminating the language barrier and can also be a greater accessibility possibility with regard to each literacy and sightedness compared to subtitles.
In right now’s put up, we share our analysis for growing voice dubbing high quality utilizing deep studying, offering a viewing expertise nearer to that of a video produced straight for the goal language. Particularly, we describe our work with applied sciences for cross-lingual voice switch and lip reanimation, which retains the voice just like the unique speaker and adjusts the speaker’s lip actions within the video to raised match the audio generated within the goal language. Each capabilities have been developed utilizing TensorFlow, which gives a scalable platform for multimodal machine studying. We share movies produced utilizing our analysis prototype, that are demonstrably much less distracting and – hopefully – extra pleasing for viewers.
Cross-Lingual Voice Switch
Voice casting is the method of discovering an appropriate voice to signify every particular person on display. Sustaining the viewers’s suspension of disbelief by having plausible voices for audio system is necessary in producing a high quality dub that helps somewhat than distracts from the video. We obtain this by way of cross-lingual voice switch, the place we create artificial voices within the goal language that sound like the unique speaker voices. For instance, the video under makes use of an English dubbed voice that was created from the speaker’s authentic Spanish voice.
Authentic “Coding TensorFlow” video clip in Spanish. |
The “Coding TensorFlow” video clip dubbed from Spanish to English, utilizing cross-lingual voice switch and lip reanimation. |
Impressed by few-shot studying, we first pre-trained a multilingual TTS mannequin based mostly on our cross-language voice switch strategy. This strategy makes use of an attention-based sequence-to-sequence mannequin to generate a collection of log-mel spectrogram frames from a multilingual enter textual content sequence with a variational autoencoder-style residual encoder. Subsequently, we fine-tune the mannequin parameters by retraining the decoder and a spotlight modules with a hard and fast mixing ratio of the variation information and authentic multilingual information as illustrated in Determine 1.
![]() |
Determine 1: Voice switch structure |
Notice that voice switch and lip reanimation is barely achieved when the content material proprietor and audio system give consent for these methods on their content material.
Lip Reanimation
With conventionally dubbed movies, you hear the translated / dubbed voices whereas seeing the unique audio system talking the unique dialogue within the supply language. The lip actions that you simply see within the video usually don’t match the newly dubbed phrases that you simply hear, making the mixed audio/video look unnatural. This may distract viewers from participating totally with the content material. In reality, individuals typically even deliberately look away from the speaker’s mouth whereas watching dubbed movies as a way to keep away from seeing this discrepancy.
To assist with viewers engagement, producers of upper high quality dubbed movies might put extra effort into rigorously tailoring the dialogue and voice efficiency to partially match the brand new speech with the present lip movement in video. However that is extraordinarily time consuming and costly, making it price prohibitive for a lot of content material producers. Moreover, it requires adjustments which will barely degrade the voice efficiency and translation accuracy.
To offer the identical lip synchronization profit, however with out these issues, we developed a lip reanimation structure for correcting the video to match the dubbed voice. That’s, we alter speaker lip actions within the video to make the lips transfer in alignment with the brand new dubbed dialogue. This makes it seem as if the video was shot with individuals initially talking the translated / dubbed dialogue. This strategy might be utilized when permitted by the content material proprietor and audio system.
For instance, the next clip reveals a video that was dubbed within the typical method (with out lip reanimation):
“Machine Studying Foundations” video clip dubbed from English to Spanish, with voice switch, however with out lip reanimation |
Discover how the speaker’s mouth actions don’t appear to maneuver naturally with the voice. The video under reveals the identical video with lip reanimation, leading to lip movement that seems extra pure with the translated / dubbed dialogue:
The dubbed “Machine Studying Foundations” video clip, with each voice switch and lip reanimation |
For lip reanimation, we practice a customized multistage mannequin that learns to map audio to lip shapes and facial look of the speaker, as proven in Determine 2. Utilizing authentic movies of the speaker for coaching, we isolate and signify the faces in a normalized area that decouples 3D geometry, head pose, texture, and lighting, as described on this paper. Taking this strategy permits our first stage to concentrate on synthesizing lip-synced 3D geometry and texture suitable with the dubbed audio, with out worrying about pose and lighting. Our second stage employs a conditional GAN-based strategy to mix these synthesized textures with the unique video to generate faces with constant pose and lighting. This stage is educated adversarially utilizing a number of discriminators to concurrently protect visible high quality, temporal smoothness and lip-sync consistency. Lastly, we refine the output utilizing a customized super-resolution community to generate a photorealistic lip-reanimated video. The comparability movies proven above will also be seen right here.
![]() |
Determine 2: Lip-Reanimation Pipeline: inference blocks in blue, coaching blocks in crimson. |
Aligning with our AI Rules
The methods described right here fall into the broader class of artificial media era, which has rightfully attracted scrutiny as a consequence of its potential for abuse. Photorealistically manipulating movies may very well be misused to provide pretend or deceptive info that may create downstream societal harms, and researchers ought to pay attention to these dangers. Our use case of video dubbing, nonetheless, highlights one potential socially helpful final result of those applied sciences. Our new analysis in voice dubbing may assist make academic lectures, video-blogs, public discourse, and different codecs extra broadly accessible throughout a world viewers. That is additionally solely utilized when consent has been given by the content material house owners and audio system.
The Alternative Forward
We strongly consider that dubbing is a inventive course of. With these methods, we try to make a broader vary of content material accessible and pleasing in a wide range of different languages.
We hope that our analysis conjures up the event of recent instruments that democratize content material in a accountable method. To reveal its potential, right now we’re releasing dubbed content material for 2 on-line academic collection, AI for Anybody and Machine Studying Foundations with Tensorflow on the Google Builders LATAM channel.
We have now been actively engaged on increasing our scope to extra languages and bigger demographics of audio system — we now have beforehand detailed this work, together with a broader dialogue, in our analysis papers on voice switch and lip reanimation.